Doctor Data: How Computers Are Invading the Clinic
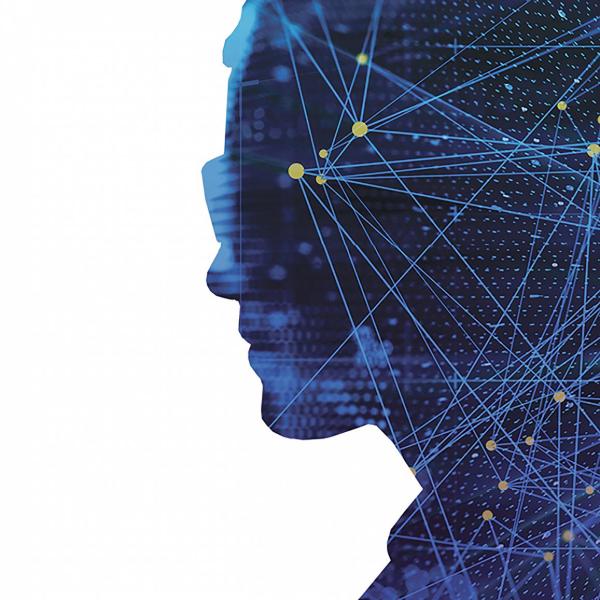
The fields of artificial intelligence and machine learning are producing computer programs that can perform tasks that previously only humans could do.
For most of their history, computers have been limited to mindlessly executing the instructions their programmers give them. However, recent advances have given rise to the intertwined fields of artificial intelligence (AI) and machine learning, which focus on the creation of computer programs that can operate independently and even teach themselves to perform specific, specialized tasks. In 2013, the online PubMed database listed only 200 research publications related to ‘deep learning,’ a new type of machine learning that has shown success for particularly difficult tasks like object and speech recognition. Just four years later, in 2017, that number exceeded 1,100.
One reason for this rapid increase is that AI and machine learning have now advanced to the point where they are beginning to penetrate fields beyond computer science, including medicine. On July 23, at an NIH workshop entitled “Harnessing Artificial Intelligence and Machine Learning to Advance Biomedical Research,” a collection of researchers came together to discuss the innovative ways they and their colleagues are taking advantage of those technologies to expand our understanding of biology and improve human health.
Among the presenters was the IRP’s own Ronald Summers, M.D., Ph.D., a senior investigator in the NIH Clinical Center’s Imaging Biomarkers and Computer-Aided Diagnosis Laboratory. Dr. Summers’ work focuses on the application of computational tools to the analysis and interpretation of medical images such as X-rays, magnetic resonance imaging (MRI), and computerized tomography (CT) scans. By leveraging cutting-edge computer algorithms, Dr. Summers aims to improve the speed and accuracy with which both computers and clinicians can detect abnormalities on medical images and use them to diagnose and treat patients. His many accomplishments include the creation of software that can predict the future size of tumors based on their past rates of growth and the design of a “virtual colonoscopy” that uses machine learning to improve the detection of colon polyps, which can be a sign of colon cancer. Dr. Summers’ team has also released to the public several datasets made up of images obtained at the Clinical Center, so that others can use them to train machine learning systems to perform useful clinical tasks. Most recently, his group released a collection of 32,000 CT scans, which could be used to create computer programs capable of detecting tumors or other abnormalities on CT images.
What follows below are some of the fascinating ideas Dr. Summers expressed during his presentation and the event’s panel discussion. You can see him speak first-hand by checking out the NIH videocast of the event. (His presentation begins at 2:22:05 and the panel discussion at 2:51:13.)
How machine learning has enhanced the ability of computers to interpret medical images
“What’s different about the machine learning era is that, in this era, hand-crafted features are less important. Hand-crafted features are things like measuring the shape of polyps or the thickness of the wall of a colon. These sorts of things require time and mathematical sophistication to figure out how to do this in an accurate way. That was time-consuming and difficult to do and required a lot of skill, but in this new era large annotated datasets are more important, and as a consequence we’ve seen more researchers entering this field and what many people have dubbed ‘democratization’ of the field. And that has led to an order of magnitude increase in the pace of research in medical image analysis.”
The aspects of electronic medical records (EMRs) that AI can use
“EMR has an incredible amount of very highly useful information. It’s not just the written reports — the progress notes, the admission history, and the discharge summary that are there — but you have all the medications, you have the vital sign information, you have all the nursing notes…Clearly, we’re not going to give up that opportunity. It’s just too rich.”
The challenge of figuring out how a computer algorithm reaches a certain conclusion
“One of the complaints about machine learning is that it’s a black box and it’s hard to understand why a machine learning system comes up with the diagnosis that it does…We can produce saliency maps showing the parts of the image that attracted the most attention by the computer system, enabling a sort of an explanation about why the deep learning system identified the particular type of disease [that it did].”
How the size of a dataset influences its usefulness for machine learning
“We started out using many small datasets. If the task is sufficiently focused, you don’t always need a huge dataset. If you’re able to localize an abnormality in an image, for example, and the range of abnormalities is relatively narrow, you don’t need a huge dataset. When you do need a huge dataset is when you’re trying to identify a diversity of different diseases. When you have a diversity of variation in normal anatomy and pathology, that’s when the requirements for more data come in.”
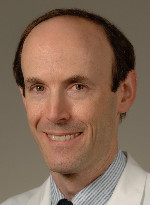
IRP Senior Investigator Ronald Summers, M.D., Ph.D.
On the potential of using AI for ‘opportunistic screening’
“The idea is that the images being acquired in routine clinical practice would be used for opportunistic screening. In the US, tens of millions of CT scans are done every year, but we don’t routinely do things like body composition analysis or quantitation of visceral fat, which has been shown to be associated with colorectal cancer and other diseases; assessment of muscle volume, which has been associated with debilitating conditions and frailty; [or] osteoporosis assessment, which has been associated with fractures of the spine and hip fractures. So by ‘opportunistic’ we mean extracting more information from what we already have. We’re just not making optimal use of the data that we already have.”
The dangers of accidentally introducing human biases into machine learning systems
“One of the risks is that those biases could actually be reinforced. One of the classic cases that’s described in the news is when a machine learning algorithm is used to determine whether an offender is likely to commit an offense when they’re released, and so decisions about whether to release offenders can be modified by these algorithms. And they’ve determined that sometimes these algorithms take into account factors like race that should be unrelated to whether that individual should be paroled or not, so it’s something we have to be very careful about.”
Applying AI and machine learning to the analysis of brain and cardiac images
“There’s a huge research community focused on brain imaging, and cardiac imaging as well. They have their own conferences, they have their own journals. There’s a lot of grant funding in brain imaging, so that’s actually a major focus area for machine learning.”
Using AI and machine learning to help clinicians choose treatments for their patients
“There is a body of research on survival prediction analysis in which different patient cohorts are compared, and that would be a means of determining which type of therapy might be more appropriate in a given patient based on clinical data. So that’s sort of a combination of diagnosis and treatment where you would say that a patient with a different set of circumstances would be likely to survive given a particular drug or treatment.”
What the future holds for teaching computers how to analyze medical images
“My expectations for the future are that the type of discoveries that can be generated by leveraging these large datasets will benefit individual patients and populations of patients through epidemiologic studies [and] by the routine integration of radiology with other clinical data, which has been relatively difficult in the past. It will also enable triage and critical result monitoring in a more rapid way than we see today. And it also has applications in global health, where there are few expert radiologists to help interpret the images but the images are getting less and less costly to produce. And, finally, there will be more automation and quantitation in radiology that will change the practice of radiology, but change has been the norm in my field so I’m not worried about it.”
Continue exploring this topic through the blog of one of Dr. Summers’ postdocs: “Challenges to Training Artificial Intelligence with Medical Imaging Data.”
Related Blog Posts
This page was last updated on Wednesday, July 5, 2023