AI Tools Provide Picture of Cervical Health
Artificial Intelligence Simplifies Cervical Cancer Screening
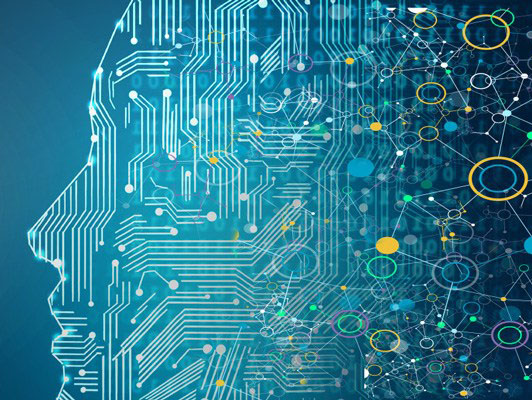
A team of IRP researchers is using artificial intelligence to create a fast, inexpensive, and easy to use method of identifying possible signs of cervical cancer using nothing more than a smartphone.
Even though cervical cancer is considered one of the most preventable forms of cancer, it remains a serious and deadly scourge for many across the world. A computer algorithm designed to quickly and easily identify pre-cancerous changes using a regular smartphone may change that.
“The point of everything that we do and have done in the last 40 years is to understand something deeply so that we can invent simple tools to use,” says IRP senior investigator, Mark Schiffman, M.D., M.P.H. To that end, he and collaborators in the National Cancer Institute (NCI) and the National Library of Medicine (NLM), in collaboration with the Global Health Labs and Unitaid, developed and are now testing a machine learning-based approach to screening for cervical cancer, with promising results.
Cervical cancer affects the tissue at the opening of the uterus. In most cases, it is caused by the human papillomavirus (HPV), which is transmitted through sexual contact. Screening programs based on Pap smears have brought down deaths from this cancer in wealthy countries, and the use of HPV tests and a preventive vaccine introduced in 2006 promise to bolster this progress. However, nearly 14,000 cases and more than 4,000 deaths from the disease still occur each year in the U.S. alone. Moreover, these statistics are much more dire worldwide, where resource-limited countries have not carried out extensive screening.
In fact, “the absolute number of cervical cancers in the world is going up,” Dr. Schiffman says. This is largely due to population aging and disparities in access to screenings and treatments. Even in the U.S., about 20 percent of women do not get cervical cancer screenings on schedule due to factors like lack of health insurance, lack of affordable or nearby health services, and social marginalization among certain groups. In addition, preventing cervical cancer currently is relatively time-consuming and expensive, requiring a medical office visit and, if results are abnormal, a biopsy and analysis by a pathologist, followed by treatment. Therefore, a simpler but still highly effective approach to screening for the disease would benefit millions of people in the U.S. and around the world.
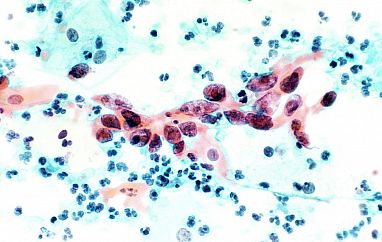
Cervical cancer cells under a microscope.
“Screening for cervical cancer is a multi-step process that's repeated many times in a woman's life in the U.S.,” explains Dr. Schiffman. “It's relatively expensive and requires technical expertise and organization.”
In parts of the world where Pap smears or HPV testing are less common, healthcare workers use a simple but less accurate screening method to look for signs of cervical cancer. They apply diluted acetic acid to the cervix and, using just a hand-held medical tool called a speculum and the naked eye, look for white areas on the cervix tissue that may indicate risk for cervical cancer. In contrast, the new approach taken by Dr. Schiffman and his worldwide collaborators, known as automated visual evaluation (AVE), uses artificial intelligence to put a fast, easy, and accurate screening tool in the healthcare worker’s hands.
“All you need is an adapted smart phone or a digital camera and a healthcare worker who can take a good cervical picture,” says Dr. Schiffman.
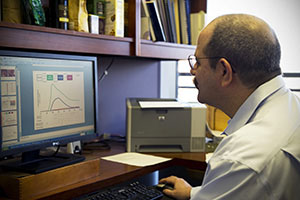
More From the IRP
Research In Action
Taking the Long View
To refine the technology, Dr. Schiffman’s team and partners are in the process of building up a data set containing thousands of digital medical images of women’s cervixes representing the continuum from healthy to cancerous. These images are used to ‘teach’ the software to recognize extremely subtle differences among the images that it can then use to classify them as healthy or unhealthy. Once the machine learning algorithm is adequately trained, a healthcare worker will be able to upload a cervical screening image to be analyzed, pixel by pixel, and labeled as healthy or abnormal and in need of further evaluation. In a study of more than 9,000 women, Dr. Schiffman’s team has already achieved good results with recognizing cervical abnormalities that could progress to cancer,1 and the approach’s accuracy will only improve as the researchers supply their algorithm with even more images to learn from.
“It can predict what we’re looking at better than humans,” Dr. Schiffman says.
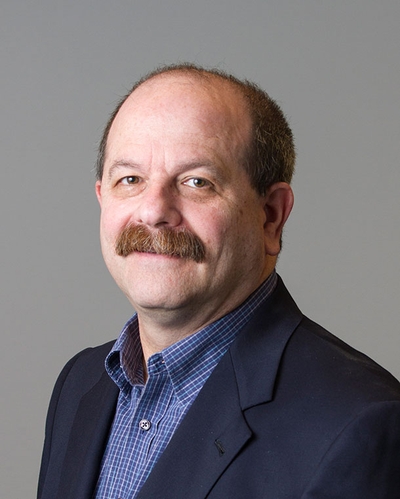
Dr. Mark Schiffman
The AI-based screening system can also be paired with new low-tech tests for HPV infection in order to boost its accuracy. Using a simple device that contains a swab and a cover, users can take a sample themselves. That sample can be rapidly tested in the clinic — it takes less than hour, during which patients can take care of other health needs, including getting the HPV vaccine for their children — or patients can mail the sample for testing.
“Self-sampling is the future, especially in cultures where tampons are used,” Dr. Schiffman explains. While acceptance is an obstacle in countries where insertable menstrual products are taboo, “you can still find good acceptability once you explain it.”
Dr. Schiffman has spent his career looking for ways to solve serious health problems, often in places with limited healthcare infrastructure, widely different cultural mores and traditions, and institutional biases. Before joining the NCI in 1983 as a staff fellow, he carried out epidemiological research in Senegal on a Fulbright Scholarship. He then traveled and worked around the world while enlisted in the U.S. Public Health Service. These experiences, as well as his interactions with members of his team who come from a wide variety of cultural backgrounds, have helped shape his worldview.
“A lot of the world lives in a way that’s quite different from the U.S.,” he says. “Many people have different views of time, risk, and their bodies. However, in terms of the fundamentals of wanting to be happy and healthy, people everywhere are the same.”
Subscribe to our weekly newsletter to stay up-to-date on the latest breakthroughs in the NIH Intramural Research Program.
References
[1] An Observational Study of Deep Learning and Automated Evaluation of Cervical Images for Cancer Screening. Hu L, Bell D, Antani S, Xue X, Yu K, Horning MP, Gachuhi N, Wilson B, Jaiswal MS, Befano B, Long LR, Herrero R, Einstein MH, Burk RD, Demarco M, Gage JC, Rodriguez AC, Wentzensen N, Schiffman M. J. Natl. Cancer Inst. 2019 Sep 1;111(9):923-932. doi: 10.1093/jnci/djy225.
Related Blog Posts
This page was last updated on Wednesday, May 24, 2023