Say Hi to AI
NIH AI Symposium Highlights Potential of New Computational Tools
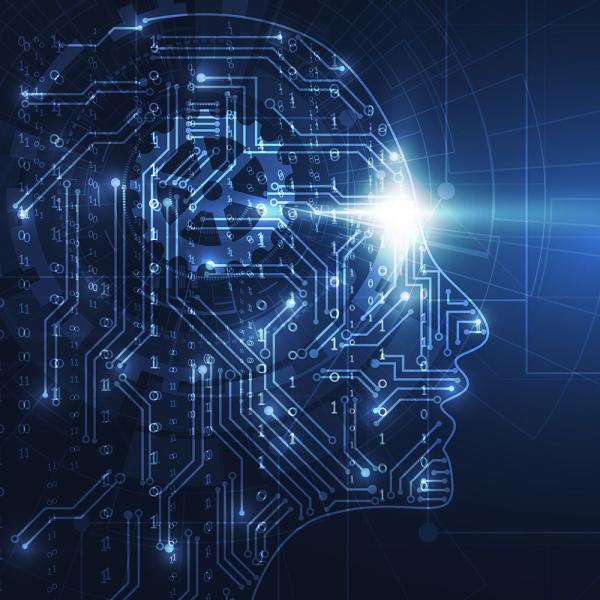
On May 17, IRP researchers came together at the first-ever NIH AI Symposium to celebrate and discuss how artificial intelligence (AI) tools are contributing to biomedical research.
The human brain is often compared to a computer. Although scientists and philosophers have long debated the appropriateness of that analogy, there’s no doubt that if our brains are computers, evolution takes its sweet time between software updates. Compare that to the rapid advancement of modern computers and it’s clear why many researchers are turning to software to assist the biological computer nature placed in their own heads.
On May 17, NIH celebrated this remarkable partnership between humans and machines with its first-ever Artificial Intelligence Symposium, a day-long event that brought together researchers from all around the IRP to share the ways their work is taking advantage of artificial intelligence (AI) and machine learning, which aims to create computers that can learn the way we do. Anyone in attendance surely came away in awe of the possibilities for how such technologies could accelerate our investigation into the mysteries of biology and the development of new medical treatments. For those who missed it, read on for a rundown of a few of the many research projects IRP researchers presented at the event.
Artificial Intelligence Accelerates Aging Research
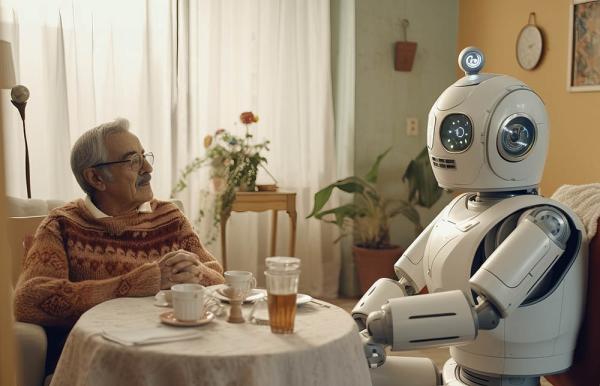
IRP researchers are using AI tools to learn more about what happens to our cells as we age.
To scientists like IRP graduate student Bradley Olinger, age is more than just a number. Rather than merely counting birthdays to assess how old somebody’s body is, Bradley and his colleagues in the lab of IRP investigator Nathan Basisty, Ph.D., want to quantify aging by measuring molecules called senescence-associated proteins, or SAPs, in people’s blood. These SAPs are thought to be somehow related to the process of senescence that gives them their name — a process that causes an accumulating cascade of problems as people age.
“Cellular senescence is a process where healthy cells lose their ability to replicate and begin inducing inflammation in the body,” Bradley says. “Studies have shown that senescence increases with age in humans across all tissues, and that it plays a role in age-related disease.”
“In recent years, a group of compounds have emerged — known as senolytics — that can selectively kill senescent cells while leaving healthy cells mostly unscathed,” he adds. “These compounds have demonstrated an ability to lower senescence burden and to partially alleviate some inflammatory diseases. While some of these compounds are drugs that were developed in labs, some of the most widely studied senolytics are natural compounds that can be found in fruits and vegetables, such as quercetin from apples and fisetin from strawberries, so the old saying ‘An apple a day keeps the doctor away’ seems to be true.”
However, Bradley and his labmates aren’t content to simply tell people to eat their fruits and veggies. Rather, by figuring out which SAPs are most closely linked to age-related disease, they hope to create a simple way to gauge the amount of cellular senescence occurring in a person’s body, allowing doctors to recommend personalized treatment plans and researchers to create new ‘senotherapeutic’ drugs that combat age-related disease by reducing senescence. Of course, like many goals in science, this is easier said than done, as there are many different SAPs and certain SAPs may be more involved in senescence in certain types of cells.
That’s where AI comes in. In the study he presented at the AI Symposium, Bradley and his IRP colleagues used machine learning to sift through a large set of SAPs in order to identify those that seem to have the biggest negative impact on human health. The researchers used data from the long-running Baltimore Longitudinal Study of Aging (BLSA) to train a computer algorithm to predict patients’ risk of future health problems based on the levels of various SAPs in their blood. After digesting the training dataset, the computer algorithm not only identified the SAPs most closely linked to age-related ailments, but it also proved its mettle at using those SAPs to predict health-related outcomes in an entirely different set of people from those in the BLSA.
“This panel of SAPs, when used to train models in the BLSA, were also able to predict clinical traits in an entirely different study, which highly suggests its biological relevance,” Bradley explains.
One day, this partnership between man and machine may lead to a world where the passage of time is not inevitably and inextricably linked to declining health.
“Identifying a small set of clinically relevant SAPs will allow us to potentially measure each individual’s senescence burden in a non-invasive way, thus identifying potential targets for senotherapeutics, and could allow us to assess the efficacy of novel senotherapeutics that arise in the future,” Bradley says.
Leveraging Large Language Models to Create “Doctor AI"
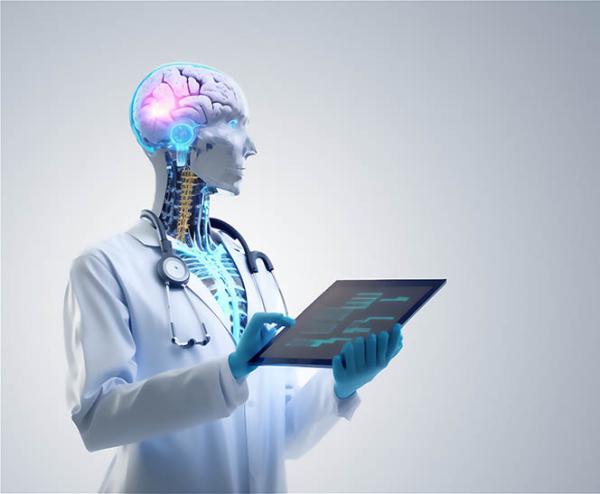
IRP research suggests that AI could one day handle some important aspects of medical care.
So-called ‘large language models’ (LLMs), such as ChatGPT, have dominated news headlines recently, and huge numbers of Americans have at least tried out the latest AI fad. As of February 2024, according to a survey by Pew Research Center, nearly a quarter of American adults say they have used ChatGPT at least once, whether to create code for computer programs, to summarize articles or presentations, or — to the disappointment of many educators — to complete their homework assignments for them.
With such a variety of uses, it’s no surprise that LLMs — machine learning models that have been pre-trained on a massive amount of data — have entered the medical field as well. For instance, IRP postdoctoral fellow Jing Wang, Ph.D., has been working on an LLM-powered program she calls “Doctor AI,” with an eye towards helping flesh-and-blood physicians better predict events that may affect their patients’ health.
“I have had the opportunity to gain experience in cutting-edge AI and machine learning over several years,” Dr. Wang says. “Large language models have shown better performance than humans in many tasks, and health care is the most promising application of AI. AI/machine learning can process large-scale datasets and learn the knowledge from the big data efficiently.”
Dr. Wang and her IRP colleagues began by feeding publicly available medical case reports about 7,000 patients into ChatGPT4. These case reports included more than 100,000 separate medical events, including adverse reactions to medications, medical diagnoses, drug prescriptions, and actions suggested by the patients’ doctors. The IRP team then used their computer program to predict what might lie ahead for a new batch of patients. They found that their AI system can look at the last 24 hours of medical history for a patient and guess what clinical events will likely occur for that patient over the next four hours with a high degree of accuracy.
“Our goal is to build an AI system which can act as an assistant of physicians and a family doctor for everybody,” Dr. Wang explains. “If the AI doctor goes public, the hospitals are going to have a physician who has seen 100 million patients and can be on call anytime. Everyone would be able to have a family doctor with many years of medical experience.”
Such an AI-powered doctor is a long-way off, but as the IRP team continues to work on their model — and as increasingly powerful LLMs continue to emerge — the possibility of a computer providing quality medical care may eventually break free from the boundaries of science fiction.
“We hope to expand the applications of our system, such as to rare diseases and cancer,” Dr. Wang says. “We also plan to invest more resources into the project, such as collecting more medical data and developing human-in-the-loop systems to collect feedback from clinicians to ensure the clinical relevance and accuracy of the system’s predictions.”
Sifting Through Saliva-Producing Cells
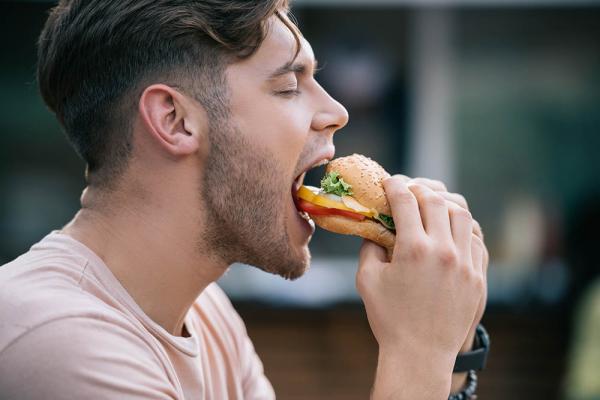
Large language models may one day help researchers correctly classify specific types of saliva-producing cells, an important step toward developing therapies for ailments that diminish saliva production.
Try wolfing down a juicy burger when your mouth is as dry as a desert and you’re not likely to have much luck. That’s because saliva plays a critical and often under-appreciated role in helping us consume food, not to mention its importance for keeping our teeth and gums healthy.
To help people whose salivary glands don’t work well, we need to learn more about them, but this endeavor is complicated by the fact that there are multiple types of cells in our salivary glands with unique characteristics. Fortunately, scientists can use two cutting-edge techniques, single-cell RNA sequencing and spatial transcriptomics, to gauge how active various genes are in cells, which helps them identify those cells, a process known as ‘annotation.’ However, trying to apply this approach to tissues in the human body that are relatively under-studied, like our microscopic ‘minor’ salivary glands, is labor-intensive and requires a high degree of expertise. In addition, even experts can disagree on what to call a cell with a certain mix of gene activity. Researchers in the lab of IRP Stadtman Investigator Blake Warner, D.D.S., Ph.D., hope to overcome those barriers using large language models (LLMs).
“Compared to the lungs, liver, or kidneys, the human minor salivary glands are not as well-studied or characterized,” explains IRP postbaccalaureate fellow Rachel Kulchar, who presented some of the Warner lab’s research at the AI Symposium. “As a result, pre-annotated datasets are not of much use since they study different tissues. Also, there is not a robust scientific literature available that will help us determine which genes are active in the cell types present in the human minor salivary glands. This makes studying salivary gland health and disease, and how different treatments affect the specific cells in the glands, difficult. Although we have experts in the lab to help confirm our annotations, we wanted to use LLMs to aid this process and compare their accuracies to that of human experts doing manual annotation.”
In the study Rachel discussed at the symposium, she and her labmates pitted four widely used LLMs — Chat GPT3.5, Chat GPT4, Copilot, and Gemini — against commonly used reference datasets of gene activity in cells, as well as annotations of single-cell RNA sequencing and spatial transcriptomics data performed by experts. Rachel and her labmates found that ChatGPT4 performed the best at this task, annotating the cell types present in the dataset with an accuracy greater than 95 percent, meaning they very closely matched experts’ annotations. Copilot and Gemini performed less well, and ChatGPT3.5 did the worst, with only about 80 percent accuracy.
Ultimately, scientists like those in Dr. Warner’s lab hope LLMs like ChatGPT4 can help them make speedier work of sifting through the secrets of our salivary glands. That will allow treatments for people with malfunctioning salivary glands to get to patients faster.
“The vast improvement from ChatGPT3.5, released in 2021, to ChatGPT4, released in 2023, highlights how these models are continually improving and underscores their potential to streamline the annotation process,” Rachel says.
Using Computer Code to Curtail COVID
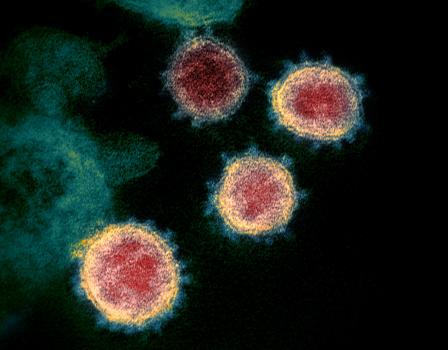
IRP researchers are leveraging artificial intelligence and machine learning to find new treatments for the SARS-CoV-2 virus responsible for COVID-19.
The explosion of interest in new artificial intelligence and machine learning tools over the past few years has coincided with intense focus on a new illness that took the world by storm during the same period: COVID-19. Naturally, scientists began applying the former to their research on the latter in the hopes that AI could accelerate the development of treatments for society’s latest viral scourge.
One such scientist is IRP postdoctoral fellow Sourav Pal, Ph.D., who is leveraging machine learning to assist a larger project called the Antiviral Program for Pandemics (APP), which focuses on creating new drugs to combat viral diseases like COVID-19. He is specifically using that AI-powered approach to speed up the identification of compounds that can inhibit a viral enzyme called Papain-like protease, or PLpro for short. PLpro allows the virus responsible for COVID-19, SARS-CoV-2, to replicate and spread inside the body, and it also helps the virus evade detection by the immune system.
“By targeting the SARS-CoV-2 PLpro enzyme, we might be able to terminate the virus's lifecycle and reduce the viral load in infected individuals,” Dr. Pal says. “Additionally, by targeting PLpro, we can potentially restore the host's immune response, allowing the body to better combat the virus. This dual action makes PLpro inhibitors particularly attractive, as they can both directly inhibit viral replication and boost the immune response.”
Dr. Pal and other members of the APP project team first used traditional ‘quantitative high-throughput screening’ (qHTS), which relies on robots, to test roughly 15,000 compounds for their ability to inhibit PLpro. This screen showed that 77 of them could inhibit the PLpro enzyme — a ‘hit rate’ of about half a percent. The researchers then used those results to train a machine learning algorithm to do the same thing via computer simulations. When the scientists subsequently unleashed that algorithm on a much larger set of compounds — about 150,000 in total — the program flagged 125 of them as potential PLpro inhibitors. Screening those 125 drugs the old-fashioned way with qHTS showed that a fifth of them could indeed inhibit PLpro. Thus, the AI-assisted drug screen had a hit rate of 20 percent — a nearly 40-fold improvement from the researchers’ initial, AI-free drug screen.
Dr. Pal and his colleagues are now working on turning the most promising PLpro inhibitors that their algorithm identified into effective drugs to combat COVID-19. With more time — and likely more assistance from artificial intelligence — scientists like them could come up with even more ways to save people from the SARS-CoV-2 virus.
“Through the utilization of AI/machine learning-based screening, we can rapidly analyze extensive datasets,” Dr. Pal says. “This approach has allowed us to efficiently explore a broader chemical space compared to the qHTS technique, whereas qHTS demands a significant amount of time and resources, making it expensive and time-consuming. In summary, the integration of AI/machine learning approaches into our study accelerates the discovery of potential candidates against the viral PLpro enzyme.”
Subscribe to our weekly newsletter to stay up-to-date on the latest breakthroughs in the NIH Intramural Research Program.
Related Blog Posts
This page was last updated on Wednesday, June 5, 2024