Supercomputing Pushes Pregnancy Research Forward
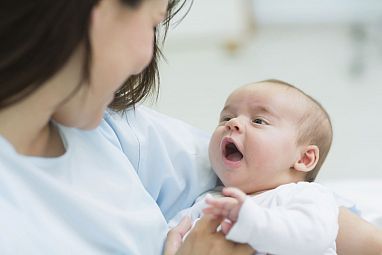
NIH’s supercomputing resources are helping IRP senior investigator Dr. Rajeshwari Sundaram to refine statistical tools that can help doctors protect the health of parents and their babies.
Virtually all parents would agree that having kids is a massive undertaking, and not just after they’re born. Many couples struggle to conceive, and each year thousands of American women experience complications when giving birth. With the help of the NIH’s state-of-the-art supercomputer, Biowulf, IRP senior investigator Rajeshwari Sundaram, Ph.D., develops and refines statistical tools that can guide prospective parents and their doctors through these challenges.
Biostatisticians like Dr. Sundaram collect and analyze huge datasets in an attempt to understand the myriad forces that influence health. Her work specifically focuses on factors that affect parents and their children starting from the time a baby is just a twinkle in his parents’ eyes. However, with a background in statistics rather than medicine, she is not interested just in determining how attributes like a mother’s age and weight affect her fertility or time spent in labor. In addition, she aims to improve the way others analyze this sort of data. By doing so, she can help create better guidelines for clinicians who work with infertile couples and pregnant women, a domain of medicine that has become more complex due to the increased prevalence of obesity, which is known to affect fertility, as well as the fact that many women are waiting longer to have children.
“It’s not just that we need newer data — we also need newer methods that can do a better job of addressing these questions,” Dr. Sundaram says. “I’m quite passionate about understanding questions surrounding pregnancy and how labor happens, and my division is a big source of epidemiological data on these topics, which puts us in a position to make a meaningful impact in this area.”
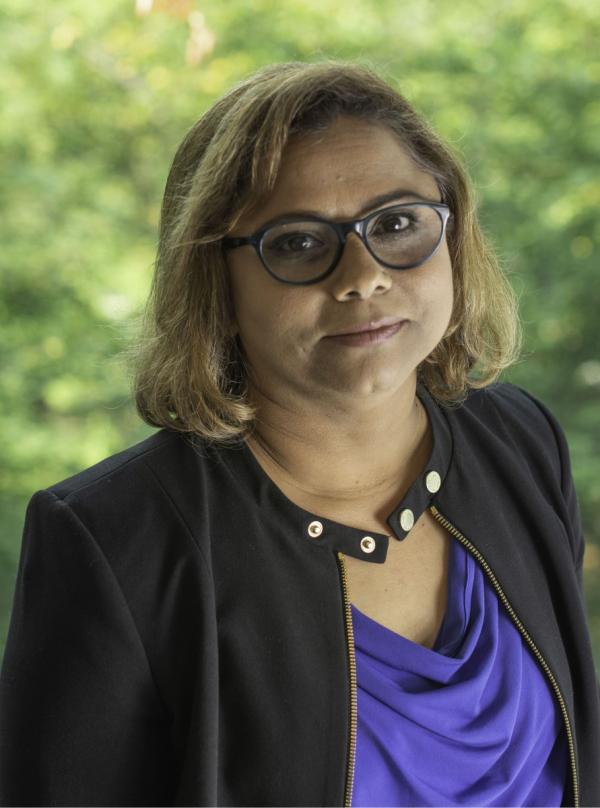
Dr. Rajeshwari Sundaram
Dr. Sundaram and her team hone the data analysis tools they develop by running simulations on Biowulf. First, they create datasets composed of numbers they generated themselves based on real data, which gives these ‘simulated’ datasets real-world applications. However, because Dr. Sundaram’s team crafts the datasets, they can imbue them with certain characteristics. For example, they might create a dataset in which two variables, such as a woman’s age and the number of attempts it takes her to get pregnant, have a certain correlation value; that is, the researchers know from the start how strong the association is between those two variables in their dataset. They then apply a statistical method they have developed for analyzing that sort of data to their custom-made dataset and see if it produces the result they expect. If the end result is close, then the statistical tool works well.
However, these tools need to perform well on datasets with widely varying properties, so Dr. Sundaram’s team runs thousands of simulations in which they apply their algorithms to many custom datasets with different characteristics. Moreover, the mathematical methods need to be not just effective but also efficient, so her research group also tracks how long it takes for an algorithm to analyze the data and spit out a result.
“That’s where Biowulf becomes very useful,” Dr. Sundaram says. “A lot of these methods are extremely computationally intensive, and when we run simulations, we do thousands of replications on various different sample sizes. It’s like running thousands of experiments at the same time.”
Recently, Dr. Sundaram has begun taking advantage of machine learning in the hopes of developing better methods of predicting infertility and identifying abnormalities that occur during labor. As artificial intelligence continues to advance, such techniques could dramatically alter the way doctors respond to the problems commonly encountered by women who are pregnant or trying to become pregnant.The statistical methods Dr. Sundaram develops have a wide range of applications. One recent project examined data on the development of young children conceived through infertility treatments.1 Because having young children is so time-consuming, many of the parents were too busy to respond to all of the surveys the investigators sent them. Dr. Sundaram’s team worried that this missing data was ‘non-ignorable,’ a statistical term meaning that missing data is directly related to what’s being measured. In this case, it could be that parents with developmentally challenged children dropped out of the study at higher rates due to the increased demands of caring for such children. Dr. Sundaram’s team created a new statistical process for analyzing datasets with these sorts of non-ignorable holes in them that performed nearly as well as the standard method but it took less time to run. Methods she has helped develop can also be applied to refining guidelines for determining when labor has slowed to a point requiring medical intervention2, as well as to the creation of predictive models that help couples alter their behavior in ways that increase their odds of conceiving a child.3
“Biowulf is critical for that work,” Dr. Sundaram says. “It doesn’t matter what project I’m working on — all my methods development is computationally intensive or uses big data, and machine learning with big data is where I see the future.”
Subscribe to our weekly newsletter to stay up-to-date on the latest breakthroughs in the NIH Intramural Research Program.
References:
[1] A Two-Step Approach for Analysis of Nonignorable Missing Outcomes in Longitudinal Regression: an Application to Upstate KIDS Study. Liu D, Yeung EH, McLain AC, Xie Y, Buck Louis GM, Sundaram R. Paediatr Perinat Epidemiol. 2017 Sep;31(5):468-478. doi: 10.1111/ppe.12382.
[2] Analysis of Gap Times Based on Panel Count Data With Informative Observation Times and Unknown Start Time. Ma L, Sundaram R. J Am Stat Assoc. 2017 Sep 26;113(521):294-305. doi: 10.1080/01621459.2016.1246369.
[3] Joint analysis of longitudinal and survival data measured on nested timescales by using shared parameter models: an application to fecundity data. McLain AC, Sundaram R, Buck Louis GM. J R Stat Soc Ser C Appl Stat. 2015 Feb;64(2):339-357. doi: 10.1111/rssc.12075.
Related Blog Posts
This page was last updated on Wednesday, May 24, 2023