Robot Superheroes to Big Data
As a child I liked robots. Growing up in Korea, I liked cartoons and movies where people were on a mission to save the world with the robots they invented, and I wanted to develop a superhero robot someday, too. While my robot isn’t yet complete, the path I followed in pursuit of my goals eventually led me to explore data analysis.
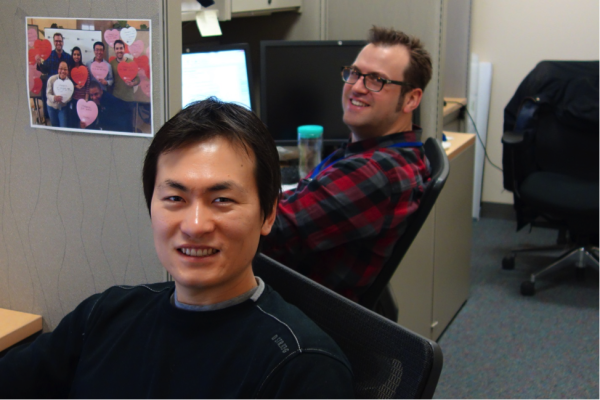
Me (front) and my fellow postdoc Holger Roth in our lab
And here I am, a postdoc at the NIH—probably the largest healthcare research institution in the world—in the Imaging Biomarkers and Computer-Aided Diagnosis Laboratory led by Dr. Ronald M. Summers. Our lab is part of the Department of Radiology and Imaging Sciences at the NIH Clinical Center.
How did I arrive here?
My first step was to study mechanical engineering when I went to college in Korea. But soon after learning about the details of robotics, I became more interested in the “brain” of the robot than its “body”—I wanted to help robots decide what to do and how to behave or move, based on what they perceive. So I added computer science onto my studies, followed by electrical engineering in Germany, which allowed me to learn about magnetic resonance imaging (MRI). MRI technology, and its potential effects on healthcare research fascinated and inspired me to pursue a Ph.D. in the U.K., researching automated analysis of patient MRI images using artificial intelligence.
While I enjoyed my time in Europe, I also wanted to experience the U.S. And I wanted the next step on my career path to be, preferably, in a large institution, so that I could learn a broader spectrum of research and science. The NIH seemed like one of the top places meeting those criteria, so I applied for a position.
What am I doing?
Here at the NIH, I analyze image and text data collected from patient care, which has been gathered in the picture archiving and communication systems (PACS) of the NIH Clinical Center over the past 10 years. “Big data” analysis can lead us to gain useful, possibly unprecedented insights in the area under study. But big data analysis is challenging too, because most data is too big to be examined manually, as well as being heterogeneous and noisy, so it is difficult to draw conclusions from the data without powerful tools and strategies.

Poster for the IEEE CVPR 2015 conference
Therefore, we use techniques that summarize the characteristics of clinical data to learn step-by-step. The first work we did on patient records of a large population was submitted to the Institute of Electrical and Electronics Engineers (IEEE) Conference on Computer Vision and Pattern Recognition (CVPR). Our research was accepted and will be presented at the conference in June.
In brief, the artificial intelligence software that our team developed can take the image of a patient’s scan and predict the “keywords” that a human researcher or doctor would associate with the image. For example, given a CT image with lung cancer, the program might generate “adenopathy”, “masses”, “lung”, or similar terms. The rate of predicted disease-related words matching the actual words in the reports’ sentences was 56%. Comparing this rate to that of other published studies attempting to describe images on the Internet (Flickr), where the rate of matching to the original user annotated text ranges from 16% to 55%, our team’s findings are very promising.
Where am I going?
In my future blog posts, I’ll share my experiences with data analysis—how to do it and some of my opinions or thoughts while doing it—starting with an automated text analysis (perhaps images too) on the previous postings of the “I Am Intramural” Blog! I’ll post some computer source code on the way too, so that the results can be reproduced and the procedures can be learned by others to be applied to other similar research purposes. Also, although I’m not yet familiar with it, I’m interested in genomics too (like many others at the NIH) and will post about genomics as I learn about it—from a data-analysis perspective.
Related Blog Posts
This page was last updated on Wednesday, July 5, 2023