Escaping the Bounds of Biology
Computational and Experimental Techniques Converge to Reshape Research
BY MICHAEL TABASKO, THE NIH CATALYST
A sea change is underway in biomedical research. Lifted by a tide of new experimental lab techniques, scientists are merging innovative technologies with synthetic methods like artificial intelligence (AI) and machine learning (ML). It’s a synergistic approach that is accelerating our understanding of human health and disease and building completely new biology to tackle difficult diseases.
Other computational tools are gleaning fresh insight from existing medical data to enhance diagnostic and prognostic methods across diverse patient populations. ML is also proving a useful partner in human genomics by finding previously unknown genetic drivers behind disease. Read on for a sample of these tantalizing technologies being used across NIH and beyond.
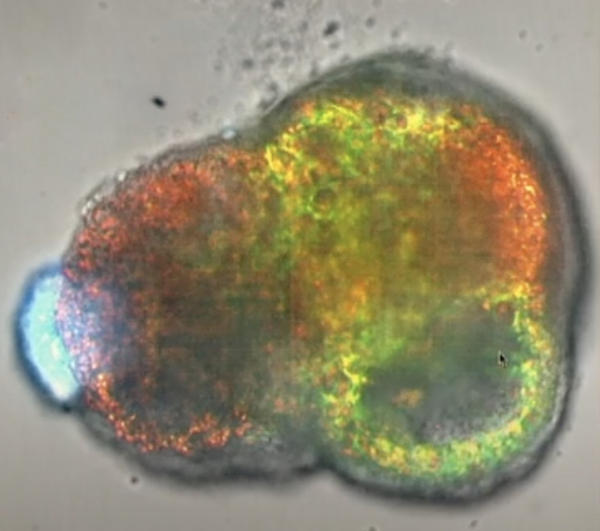
CREDIT: WENDELL LIM, UCSF
Lim and colleagues designed synthetic organizer cells that directed the assembly of a heartlike structure that beats, complete with a cavity and peripheral vasculature. Lim serves as director of UCSF’s Center for Synthetic Immunology, an NIH IOTN-i3 Center supported by NCI, NIA, and NIBIB.
Rewiring cells
Consider an immune cell engineered to kill cancer by recognizing multiple antigens; or cells reprogrammed to deliver drugs to specific sites in the body.
These ideas are the realm of generative biology, according to pioneer Wendell Lim, professor at the University of California at San Francisco (UCSF). On March 13, Lim delivered a Wednesday Afternoon Lecture Series talk titled “Generative Biology: Learning to Program Cellular Machines.” With the help of ML techniques that comb through genomic data to model how a reengineered cell might behave, Lim and his team can create purpose-built cells to carry out specific therapeutic tasks to help fight against some cancer, autoimmune, and neurodegenerative conditions.
In contrast to small-molecule drugs, which can result in whole-body side effects, Lim likens his lab’s rewired cells to powerful machines that can be designed to execute precise, targeted effects.
One such technology is synthetic notch (synNotch) chimeric antigen receptor (CAR) T cells. In mice, Lim’s team showed how these cells can be programmed to recognize a combination of multiple antigens unique to a particular tumor, and then activate only in that target tissue. For example, they can target glioblastoma in the brain by creating a “controlled blast radius” whereby the tumor cells are killed while healthy tissues elsewhere are largely spared (PMID: 33910979). By tweaking the combination of antigens to target, synNotch can be navigated toward other locations in the body or other diseases by acting as a cellular GPS to deliver a therapeutic payload precisely where it is needed.
Synthetic cell-adhesion molecules are another intriguing technology that uses the existing rules of biology to create something new. They can be customized to orchestrate cells into self-organized assemblies that resemble natural tissue or even completely novel ones (PMID: 36509107). In one example, Lim’s team showed how rewired cells assembled and drove stem cells to differentiate into a heartlike structure that beats, complete with a central cavity and peripheral vasculature. He hints that such choreographed behaviors might someday be harnessed to reconstruct damaged nerves or organs.
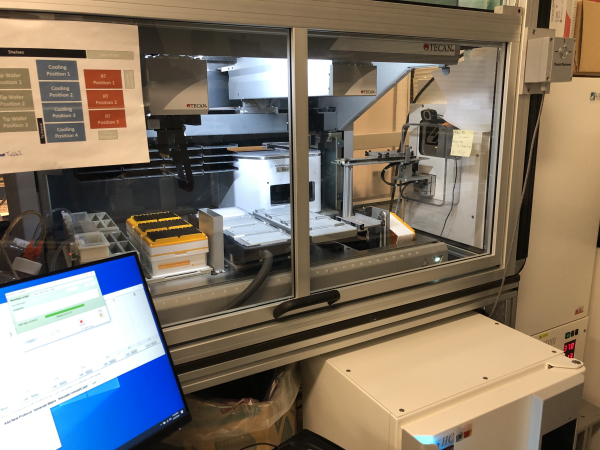
CREDIT: GRÉGOIRE ALTAN-BONNET, NCI
At NCI’s Laboratory of Integrative Cancer Immunology, an automated robotic platform tests T cells on samples from mouse studies and clinical trials. Then, supervised machine learning helps model the unfolding immune dynamics to inform the design of new tumor-specific T cells.
Optimizing T cells at NIH
Constructing computational models of the immune response can serve as a roadmap to design better immunotherapies against tumors. The lab of Grégoire Altan-Bonnet, senior investigator at NCI’s Laboratory of Integrative Cancer Immunology (LICI), created a robotic platform that streamlines and automates how CAR T cells are tested on samples from both mouse studies and clinical trials. In close collaboration with Paul François’s group at the Quebec Artificial Intelligence Institute (known as Mila), the LICI team uses supervised ML to analyze the unfolding immune dynamics in those samples and uses that data to create models of how immunotherapies might work. That new platform discovered six classes of antigens that elicited distinct T cell responses (PMID: 35587980).
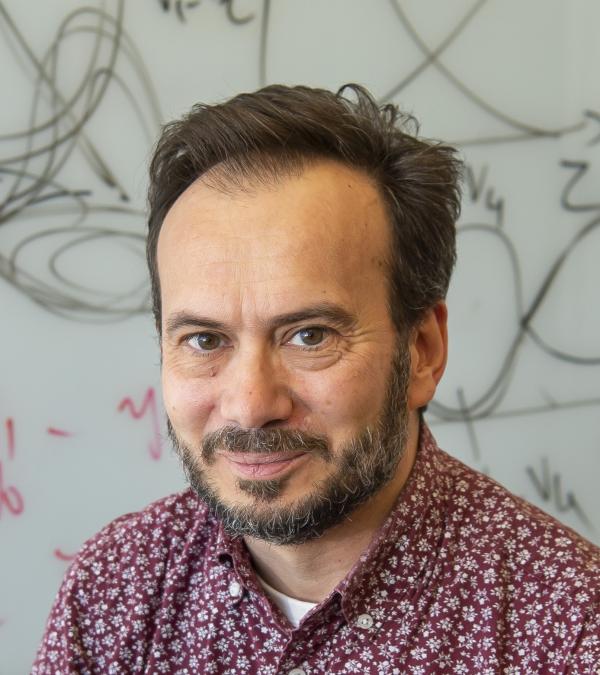
CREDIT: GRÉGOIRE ALTAN-BONNET, NCI
Grégoire Altan-Bonnet
Importantly, LICI’s immune models are tuned to pick up on signals coming from healthy tissue as well as diseased tissue. That method better reflects the complex immune environment and ideally results in safer therapies when a drug moves to clinical trials. “At this stage, we know it is more critical to actively shut down the response in the healthy tissue while boosting your response in the tumor,” said Altan-Bonnet, who tempers the exuberance currently surrounding AI and ML with cautious optimism. “We must do it right. There’s a lot to be done in terms of doing the proper ML [that] connects to the proper biology and being able to use it.”
Better drug screening platforms
Once a shiny new therapeutic is created, testing it for safety and efficacy begins. That’s where wet-lab programs such as NCATS’ intramural 3D Tissue Bioprinting Laboratory led by Marc Ferrer can come in. The lab is a cross-disciplinary group that biofabricates in vitro complex cellular models for drug discovery and development. The hope is that these models are better at predicting how effective or toxic a drug may be in humans compared with simple cell lines. “In the body, different cells talk to each other, and these models mimic how cells behave in human tissues and organs, and therefore drugs should respond more like in the body,” said Ferrer.
The bioprinting process begins with mixing a hydrogel of the right viscosity with human cells. For example, a mixture of keratinocytes and fibroblasts are used to make a human skin model. Software is used to design the shape of the tissue, which is then crafted by extrusion of the gel into specific layers of different cell types that will soon resemble real tissue. Nature then takes over and the model matures, secreting other components such as collagen and other support proteins in the case of skin tissue.
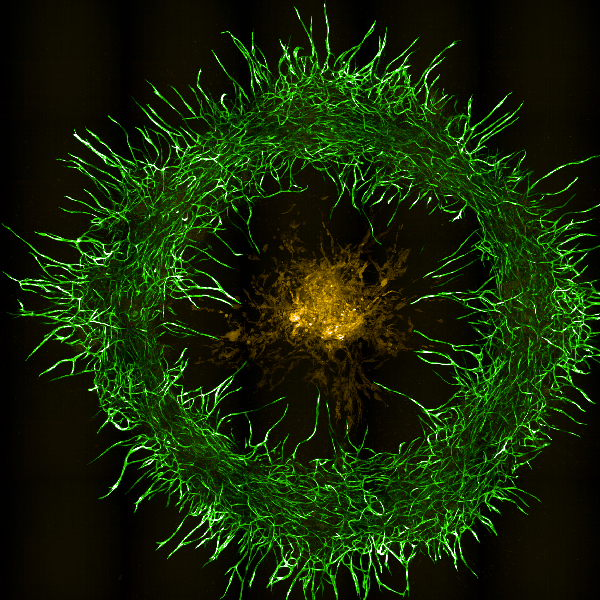
CREDIT: YEN-TING TUNG, NCATS
Shown here is a 3D bioprinted neurovascular unit tissue model surrounding patient-derived glioblastoma (GBM) cells using human brain microvascular endothelial cells (green), pericytes, astrocytes, and patient-derived GBM cells (yellow).
Once Ferrer’s team validates the healthy model, they can make a diseased one and then test drugs on it. The approach can complement or provide an alternative to animal models and has broad application. For example:
- A bioprinted model of the retina was used to test a clinically approved antibody for age-related macular degeneration (PMID: 36550275).
- A disease model of atopic dermatitis in human skin successfully showed how JAK inhibitors reversed the disease state (PMID: 32059197).
- A 3D bioprinted neurovascular unit model demonstrated how glioblastoma tumors grow in a brainlike environment and can be used to test anticancer drugs (PMID: 38394389).
“Establishing validity and predictability of these tissue models is very important,” said Ferrer, who added that these models are used in preclinical studies to allow investigators to look closely at the mechanisms underlying both the disease and how a drug might work for that disease.
How NIH researchers use bioprinting to create eye tissue
AI-powered predictions
Back in the synthetic space, investigators are using AI to decipher big data and unearth clinically significant insights. “We now have computational ways to predict causal mutations for all complex diseases,” said Ivan Ovcharenko, senior investigator at NLM, whose research spans Alzheimer’s disease, ovarian cancer, and glaucoma. “This opens doors for more diagnostics, screenings, and studies to find new drug targets,” he said. His group uses deep learning methods (DL) to computationally mutate every single disease-associated nucleotide in a process known as “in silico mutagenesis” and predict which ones are causative. Then, they collaborate with other labs that confirm those digital findings using biochemical techniques.
In one such example, a DL method applied to a genome-wide association study of type 2 diabetes found nearly triple the number of known causative genes in pancreatic islet cells compared with traditional methods (PMID: 37603758).
Ongoing work at Ovcharenko’s lab is using AI to identify silencers in the human genome. Silencers are compact clusters of noncoding genetic material that act to shut off gene expression in nearby coding regions. They act like an architect to trim the activity of enhancer regions. Without them, a gene can be expressed somewhere it should not and lead to carcinogenesis or other diseases.
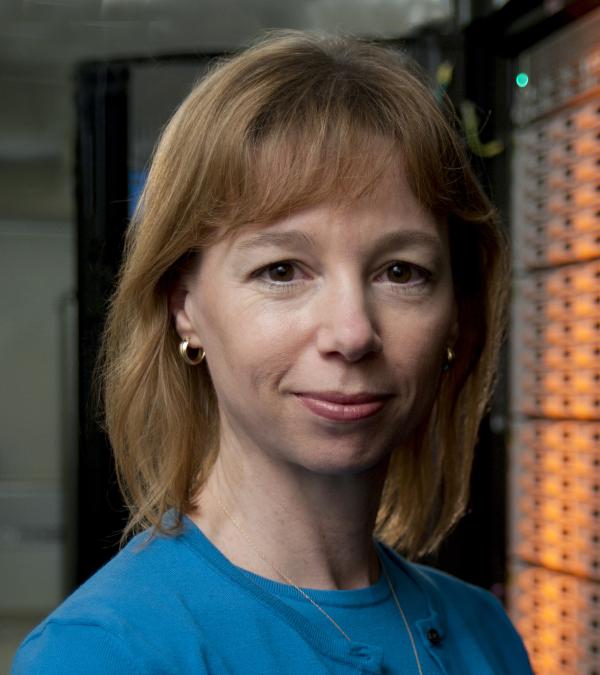
CREDIT: NHGRI
Laura Elnitski
Validating those computational predictions on real biology is the next step. Scientists such as Laura Elnitski, senior investigator at NHGRI, and Ben Afzali, a Stadtman investigator from NIDDK, then do the wet-lab work using biochemical techniques that analyze how genes express from in vivo and in vitro samples after CRISPR modification.
Work by the Elnitski group showed that silencers do indeed influence gene expression in specific tissues (PMID: 30886051), and ongoing studies are testing how alterations in genome regulation could cause B-cell lymphomas or ovarian tumors. The computational models and lab experiments are aligning. “The validation testing tells us the models are working,” she said. “Predictions from integration of data reveal dynamic biological processes.”
An upcoming collaborative study from those labs aims to demonstrate that large clusters of silencers are a critical component of cellular development and disease regulation.
“I think this is going to have a huge impact on our understanding of genetic predisposition to diseases,” said Richard Scheuermann, scientific director at NLM.
Interested in more AI? Learn about some other ways in which investigators at NLM are conducting AI-augmented research.
- Watch how Jeremy Weiss, tenure track investigator and lead of the Care Health and Reasoning Machines lab, uses computational methods to sort through clinical data and stratify patient risk for certain conditions.
- ML tools are being used to interpret imaging data more accurately on X-rays, computed tomography, ultrasounds, and magnetic resonance scans. Watch how Sameer Antani, tenure track investigator at the Computational Health Research Branch, develops ML tools that can predict what early lesions will develop into more serious conditions.
- In addition to interpreting medical images, watch how Zhiyong Lu, senior investigator at the Computational Biology Branch, develops AI techniques that help researchers keep up with the rapid growth of biomedical literature and find the data that matter most to them.
Learn more about how NIH is using AI to dig deeper into DNA differences in the latest SciBites episode
And don’t miss the NIH Artificial Intelligence Symposium, Friday, May 17, in Masur Auditorium, Building 10.
This page was last updated on Friday, May 3, 2024