Keeping the "I" in DEIA for AI, ML
Keeping Diversity, Inclusion Top of Mind When It Comes to AI, ML in Health Care
BY TAYLOR FARLEY, NIAID
In 1955, John McCarthy, a computer scientist then at the Massachusetts Institute of Technology (Cambridge), coined the term “artificial intelligence” (AI), creating language for the concept of using computers to mimic the capabilities of humanity’s greatest evolutionary asset—the mind.
Today, the future McCarthy and his colleagues envisioned is upon us with all its promise and perils. In health care in the United States, this technology has already reached 78% of patients. While mostly limited to drafting physician notes or providing care insights, AI is being eyed as the next-generation diagnostic tool.
Despite this potential, experts also warn that AI and machine learning (ML), if not properly vetted, could inadvertently amplify health disparities.
Bias in, bias out
Like human intelligence, AI, and thus ML, require the study of data entered into a system. Just as humans read to gain knowledge, so too does AI. That knowledge then can be used to train machines to consume large datasets and use those datasets to create predictive models on which we base our science.
A job that would take the human mind days, weeks, months, or even years to compute could take a properly trained AI or ML model just moments. But in their elemental form, AI and ML are math, not mind, and predictions are based on the study material supplied by humans, which comes with biases.
“The techno-chauvinistic perspective tells us that AI or computational solutions are ‘objective’ or ‘unbiased’ or ‘superior,’ but it quickly becomes clear that the problems of the past are reflected in the data,” said Meredith Broussard, a data journalist and New York University professor. “The problems of the past are things like discrimination, racism, sexism, ableism, structural inequality, all of which unfortunately occur in the world, and all of these patterns of discrimination are reflected in the data we have used to train these systems.” Broussard delivered a lecture in March, titled “How Can Cancer Help Us Understand Algorithmic Bias” as part of NLM’s Science, Technology, and Society Lecture series, during which she highlighted various ways AI and ML can exacerbate prejudice by continuing to make predictions and suggestions based on past patterns.
Similarly, Marzyeh Ghassemi, an assistant professor at MIT, specializes in the health of the machine learning algorithms and conducts research identifying how easily this technology can inadvertently exacerbate preexisting biases in medicine.
Watch Ghassemi [beginning at time stamp 52:21] explain how biases exist within electronic health records during an advisory council meeting at NIEHS.
Ghassemi’s group trained a model to identify whether a chest X-ray had concerning signs of disease that would require further evaluation (PMID: 34893776). The goal was to train the system to assist with triaging patients—can the patient return home, or do they require priority care?
When trained on three publicly available datasets, including one from the NIH, the model disproportionately misdiagnosed female, Black, and Hispanic patients, those under 20 years old, and those with Medicaid insurance, an imperfect proxy for socioeconomic status. For individuals with intersectional identities, these disparities were even more pronounced. Meaning, if this tool rolled out in emergency rooms today, some patients would be sent home without proper care.
Concerning trends of misdiagnosis for marginalized communities are widespread in American health care today. At the precipice of the new age of medicine, we have an opportunity to correct the shortfalls of the past, but it means we must move forward with intention.
NIH efforts to diversify data
Improving these systems will require training data that are more diverse, and the NIH has already established programs to tackle this ongoing limitation.
One NIH-partnered initiative aimed at expanding heath databases through purposeful inclusion of individuals from underrepresented or marginalized communities is the All of Us Research Program. Another effort, the NIH Artificial Intelligence/Machine Learning Consortium to Advance Health Equity and Research Diversity (AIM-AHEAD) Program aims to address inequities in AI and ML research and application through four areas: partnerships, research, infrastructure, and data science training.
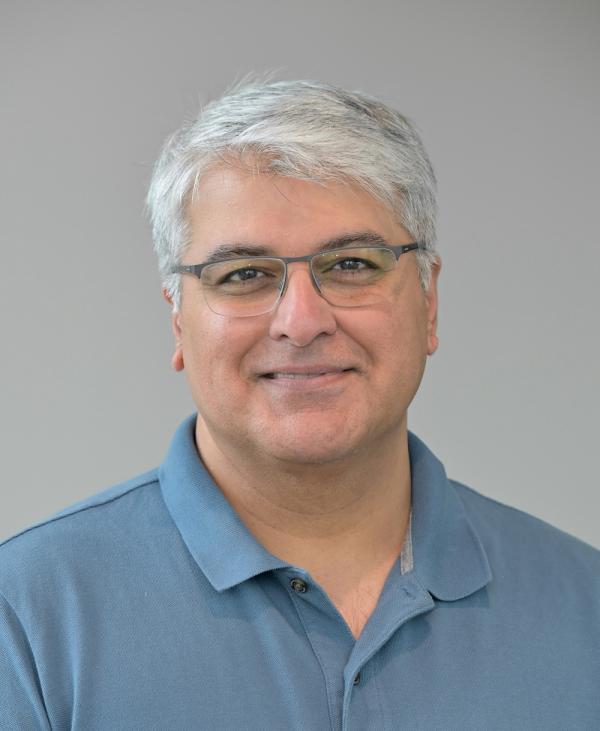
CREDIT: NLM
Sameer Antani
Sameer Antani, a tenure track investigator in the Computational Health Research Branch at NLM, conducts research on improving the performance and reliability of novel image-based and multi-modal AI and ML for various diseases. He suggests greater effort should be devoted toward minimizing apparent hubris in the technology and enabling acknowledgment of uncertainty. For example, designing the AI to project its degree of confidence in predictions, based on the training data characteristics, could help improve collaboration between the technology and humans, helping it actively learn to address the gaps, and reduce the potential for bias over time.
“Through appropriate development of this technology, we have an amazing opportunity, a second chance, to correct disparities and biases of the past, and improve health care for all,” Antani said.
As with any novel technology, it can be enticing to run in the direction of innovation and ignore the potential negative consequences. But when it relates to the health of our world, we must continue to ask ourselves: Is it helping us all equally in the process?
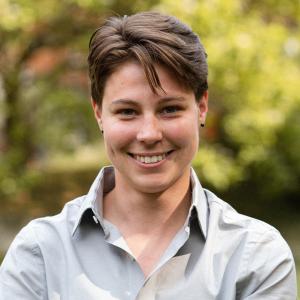
Taylor Farley is a postdoctoral fellow studying innate-like immune responses to the microbiota in the gut during homeostasis and inflammation. When not in the NIAID lab, she enjoys playing guitar, rock climbing, and cheering on The Roommates during their Sunday LGBTQ+ Stonewall Kickball season.
Rall Lecture Features Famed Father of the Internet
BY MEAGAN MARKS, NIAAA
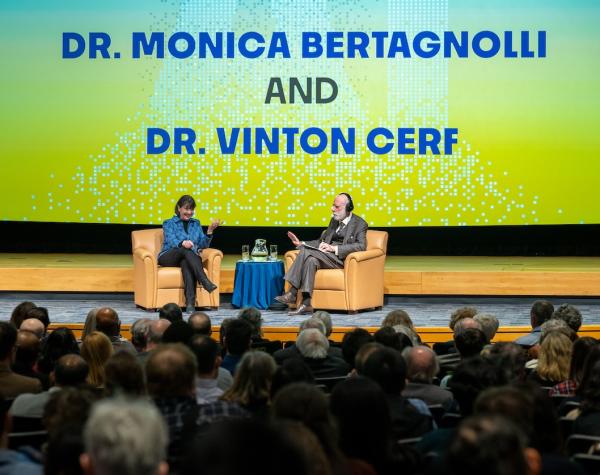
CREDIT: CHAICHI CHARLIE CHANG
The annual J. Edward Rall Cultural Lecture featured Vinton Cerf, oft coined a “father of the internet,” who delighted hundreds of attendees in Masur Auditorium on March 19 with his humor and quick wit.
Cerf sat down with NIH Director Monica Bertagnolli for a fireside chat focused on a matter top-of-mind for NIH’s new leader: The future of AI and ML in health care.
The duo discussed how recent advances in AI might influence biomedical research and the future of health care delivery. They answered NIH audience questions that broadly asked:
- Can AI make health care truly evidence-based?
- How should providers preserve, protect, collect, regulate, and diversify the health data of patients?
- What are the most practical and reliable uses of large language model technology such as ChatGPT (if any) in medicine?
Answers explored how AI might aid in 24/7 patient monitoring and how ML could one day lead to personalized medicine and personalized treatment; and the pair estimated the immense potential of electronic health records.
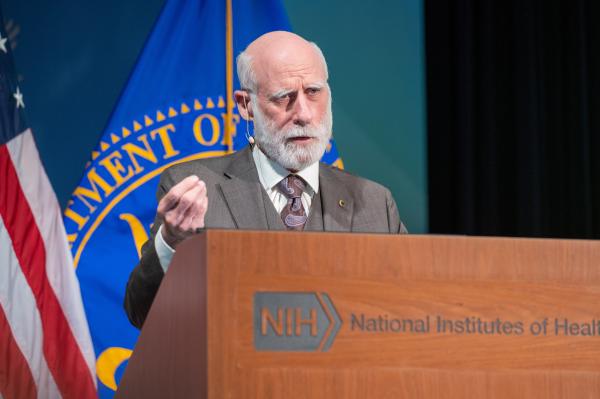
CREDIT: CHAICHI CHARLIE CHANG
Vinton Cerf, a father of the internet, was the 2024 J. Edward Rall Cultural Lecture featured speaker. The Rall lecture is an installment of the Wednesday Afternoon Lecture Series (WALS).
“I hope you recognize what an opportunity NIH has to improve the state of health care with the kind of research that you are doing and the attempt to exercise these powerful computing tools that are emerging,” Cerf said to the audience in his closing remarks. “You have an opportunity to make a lot of progress…and if I were in your shoes, I would be sitting here thinking, ‘Boy, do we have a lot of work to do and a huge amount of opportunity to do good.’”
Experience the full discussion and Cerf’s intelligently detailed responses by viewing the lecture in its entirety at https://videocast.nih.gov/watch=54305.
This page was last updated on Monday, May 6, 2024