2019 Research Festival: Plenary III
Celebrating Cutting–Edge Technologies
If you asked intramural researchers what their favorite part of working at NIH is, many would tell you it’s the availability of cutting-edge technology. Four scientists shared their stories.
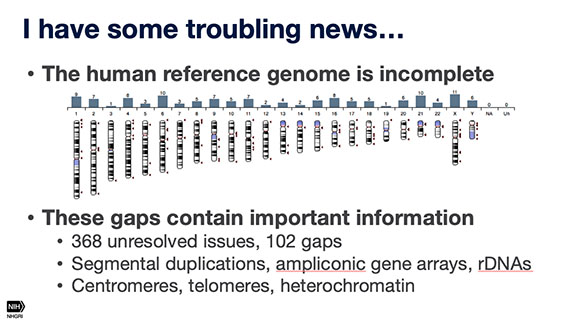
A nanopore-sequencing technique shows promise, for completing the human genome, Adam Phillippy (NHGRI) told audience members at the Research Festival. Although the scientific community reveled at the 2003 announcement that the entire human genome had been sequenced, it is really about 95% complete, given the technology available at the time, said Phillippy.
The first speaker, Adam Phillippy, who’s head of the Genome Informatics Section in the National Human Genome Research Institute, talked about a nanopore sequencing technique for completing the human genome. The scientific community reveled at the 2003 announcement that the entire human genome had been sequenced, but it is really only 95% complete given the technology available at the time. Typically, to avoid being overwhelmed, DNA-sequencing machines chop up copies of the 23 pairs of chromosomes (some 3 billion base pairs) into chunks containing a few hundred base pairs. The overlaps are matched up and the chunks assembled into the correct sequence. “But like a jigsaw puzzle, the smaller the pieces, the harder the puzzle is to assemble,” said Phillippy, and the more likely there will be gaps in the sequencing.
Phillippy’s lab is applying a newer technique, called real-time nanopore sequencing, to solve this problem. By pulling a single strand of DNA through a protein nanopore (modified from a transporter protein in Escherichia coli), the sequencer can produce DNA-sequencing reads up to one million base pairs long. He hopes to produce reads long enough to accurately cover difficult-to-sequence regions of the genome, thus filling in the gaps and truly completing the Human Genome Project. While completing the human genome offers a monumental advantage to human research worldwide, the mere availability of nanopore sequencing provides scientists a new tool to tackle difficult genomic questions across all species.
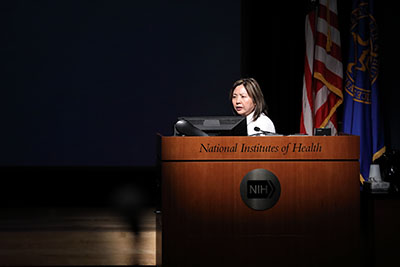
CREDIT: CHIA-CHI CHARLIE CHANG
The current treatment for chronic granulomatous disease (CGD) is bone-marrow or stem-cell transplant but it’s difficult to find suitable donors. Scientists are also exploring gene therapy—using a retroviral vector to insert modified genes into a patient’s cells—shows some promise but offers only temporary improvement. Elizabeth Kang (NIAID) is testing a lentiviral vector—which can enter both dividing and nondividing cells (standard retroviruses can only enter dividing cells)—as a better gene-therapy treatment for CGD.
The second speaker, physician Elizabeth Kang (National Institute of Allergy and Infectious Disease, NIAID), shifted the focus from reading the genome to modifying it. Kang heads up the Hematotherapeutics Unit in NIAID’s Laboratory of Clinical Immunology and Microbiology and frequently conducts clinical trials. Her current projects focus on chronic granulomatous disease (CGD), a primary immunodeficiency disease in which patients have defective neutrophils unable to fight off specific infections. These neutrophils also form granulomas, hence the name of the disease, and patients are also prone to autoimmune problems such as inflammatory bowel disease.
The current treatment for all types of CGD is bone-marrow or stem-cell transplantation, but it can be difficult to find a suitable donor and there’s a risk of tissue rejection as well as graft-versus-host disease, in which the donor graft attacks the normal tissues of the patient. Gene therapy—using a retroviral vector to insert modified genes into a patient’s cells—is being explored, but so far it offers only temporary improvement.
Kang’s lab is testing a different type of retrovirus vector called a lentiviral vector, which can enter both dividing and nondividing cells (standard retroviruses can only enter dividing cells). The researchers found that using this vector combined with a white-cell-specific promoter for the NADPH oxidase enzyme resulted in an increased production of the non-mutated protein in both mouse and human cells. Their clinical trials in patients with the X-linked form of CGD are showing that the patients have high nicotinamide adenine dinucleotide phosphate oxidase production that remains relatively high even two years post-treatment. Kang is conducting other studies to determine how to further improve genetic therapies for the X-linked form as well as other types of CGD.
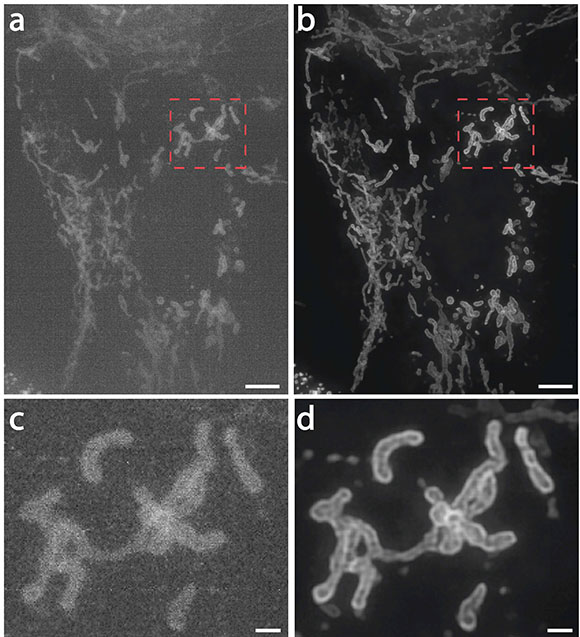
CREDIT: UNPUBLISHED DATA, JIJI CHEN (AIM), YIJUN SU (NIBIB), HARI SHROFF (NIBIB, AIM), HOYIN LAI (DRVISION), HIDEKI SASAKI (DRVISION), LUCIANO LUCAS (DRVISION).
Hari Shroff (NIBIB) described how his team is applying deep-learning techniques to achieve better fluorescence microscopy. Shown: Mitochondria in live human bone osteosarcoma epithelial cells (U2OS line): (a) imaged on a high-speed structured illumination microscope at low power; (b) Artificial intelligence algorithms were used to greatly restore signal, contrast, and resolution; (c, d) show higher magnification views of red dotted rectangular regions in a and b. NOTE: Scale bars are 5 micrometers in a and b and 1 micrometer in c and d.
The next speaker delved into the world of artificial intelligence. Senior Investigator Hari Shroff (National Institute of Biomedical Imaging and Bioengineering, NIBIB) described how his team is applying deep-learning techniques to achieve better fluorescence microscopy. Deep learning is a type of machine learning in which computer systems automatically learn from experience, without being explicitly programmed, by analyzing data to look for patterns, determining complex statistical structures that identify specific features, and finding those same features in new data. In deep learning, massive amounts of data and powerful computers train large and complicated artificial neural networks.
Shroff’s lab is applying deep learning to improve and augment fluorescent-microscopy images. Normally, taking high-resolution images requires applying a strong laser to a sample; the laser quickly bleaches the sample of its fluorescence. The deep-learning neural network, however, can read lower-level fluorescence taken over a longer time and then produce a high-resolution image by predicting it from the data. Shroff has been using this technology in conjunction with the Trans-NIH Advanced Imaging and Microscopy (AIM) facility, with the eventual goal of disseminating the technology so that NIH researchers can use the microscopes and computer algorithms to make high-resolution and long-term fluorescent images. A computer that thinks more like a human can be an unsettling notion, but there is no denying its usefulness, and Shroff’s team has shown its reliability in predicting high-resolution images at very low illumination.
The final speaker, Hannah Valantine, is a senior investigator (National Heart, Lung, and Blood Institute) as well as NIH’s Chief Officer for Scientific Workforce Diversity. Her research focuses on finding better ways to predict and diagnose heart-transplant rejection, a common problem in people who’ve received the organ. Current methods to detect rejection involve painful and invasive biopsies of the donated heart tissue. A patient once told her, “Dr. Valantine, you gave me a heart, then you take it away, bit by bit. It’s not fair.”
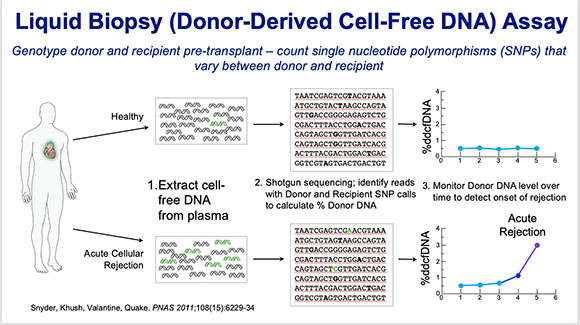
Hannah Valantine (NHLBI) has developed a non-invasive liquid biopsy method as an alternative to the physical biopsy for predicting whether a transplanted heart is in danger of being rejected.
Valantine has developed a noninvasive liquid-biopsy method—a simple blood test for cell-free DNA (cfDNA)—as an alternative to the physical biopsy for predicting when a transplanted heart is in danger of being rejected. The liquid-biopsy technique was first developed at Stanford University (Stanford, California) and involved detecting and sequencing fetal-cell-free DNA from a mother’s blood for prenatal diagnosis of genetic abnormalities such as Down syndrome. Valantine developed a similar technique to predict organ rejection in heart-transplant patients and found that rapidly increasing concentrations of donor cfDNA predicted rejection.
She also set up the Genomic Research Alliance for Transplantation (GRAfT) among the NIH and multiple hospitals and donation centers across the region. GRAfT studies have shown that these cell-free DNA tests are reliable and reproducible and act as good predictors of long-term outcomes. Valantine hopes the technology will prove a noninvasive and valuable tool for detecting rejection and allowing early treatment for heart-transplant patients.
To watch the videocast of Plenary Sessions II and III, go to https://videocast.nih.gov/launch.asp?28720.
This page was last updated on Wednesday, March 30, 2022