Brain Data Predicts Alcohol Disorder Symptoms
Study Results Could Help Improve Treatment for Alcohol-Related Problems
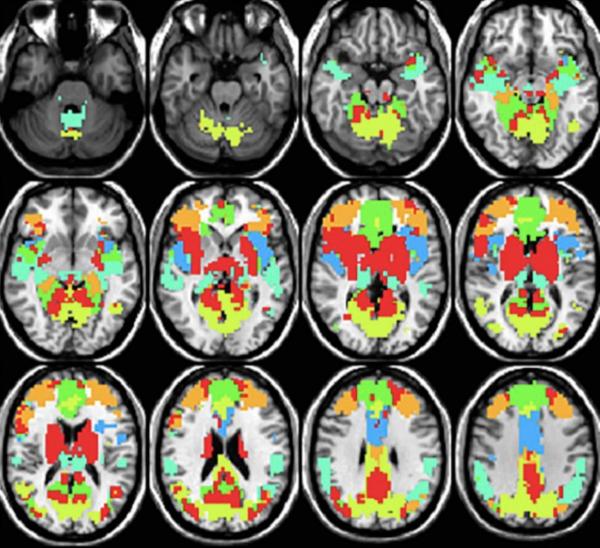
IRP researchers discovered that the interactions of different brain regions when the brain is not engaged in a task could be used to predict the severity of individuals’ alcohol-related problems.
Your brain is always busy, even when you’re not thinking about anything. Scientists believe the way brain cells communicate with one another when the brain is in that ‘resting state’ might differ in individuals with certain diseases. In a recent study of this idea, IRP researchers found that resting state brain activity could effectively predict the severity of alcohol-related problems.1
While most people can consume alcohol without it dramatically reducing their quality of life, those with alcohol use disorder (AUD) struggle to control their drinking and experience significant repercussions from it. An estimated 16 million Americans have AUD, but fewer than one in ten receive treatment. Moreover, current treatments are sometimes not effective or yield only small benefits.
Some scientists are attempting to solve these problems by comparing the brains of people with AUD to those without the illness. The IRP’s Reza Momenan, Ph.D., is among the relatively few researchers studying how the brain behaves differently in people with more severe AUD symptoms compared to those with less serious symptoms. In his new study, Dr. Momenan and his team examined the brains of people with alcohol-related problems using several neuroimaging techniques in order to identify the brain changes that most strongly relate to the severity of a person’s struggles with alcohol.
“The goal was to capture the contribution of each of these modalities to provide a more sensitive and comprehensive index of severity,” Dr. Momenan explains. “We are trying to provide a quantitative and objective measure of the state of the disease.”
Participants in the study first filled out a standard questionnaire called the Alcohol Use Disorders Identification Test (AUDIT) that measures problematic drinking behaviors and their consequences. The participants then underwent MRI scans that analyzed the physical structure of their brains as well as how active different parts of their brains were during two different tasks. In addition, the researchers collected ‘resting state connectivity’ data, which measures the extent to which different parts of the brain communicate with one another when a person is not doing anything. Past research had grouped those brain regions into various networks, and the activity within them depends on what a person is doing. For example, the brain structures in the executive control network talk to one another when a person is making plans or exerting self-control, while the default mode network is active only when people are not thinking about anything at all.
After completing the scans, the IRP researchers fed the data from 59 of their participants into a machine learning algorithm to create several mathematical models that related the various neuroimaging measures to the participants’ scores on the AUDIT questionnaire. Three of the models took into account only one type of the neuroimaging data, while another included only demographic information like age and gender. A final model took into account all this information.
Finally, the researchers had each of the models attempt to use the data collected from a separate set of 24 participants to predict their AUDIT scores. In the end, the model that included only the resting state connectivity data yielded the best predictions and identified several brain networks that work differently in individuals with more severe AUD symptoms compared to those with milder symptoms.
“These alterations point to compromises in cognition, decision making, impulse control, and compulsive behavior in those with more serious AUD symptoms,” Dr. Momenan says.
Despite its successes, the resting state connectivity model only accounted for a third of the variation in AUD symptoms among the participants, so future studies that include more individuals will attempt to identify other factors related to AUD symptom severity. Once enough data has been gathered, mathematical models like those Dr. Momenan’s team created could prove extremely useful to clinicians.
“The ability to use neural data to indicate AUD severity can be beneficial in medical settings to understand disorder severity without depending on self-reports,” Dr. Momenan says. “This, in turn, will provide an index of risk prediction, disease progress, treatment efficacy, and recovery. Accordingly, the clinicians will be further enabled to plan and implement the most suitable course of action.”
Subscribe to our weekly newsletter to stay up-to-date on the latest breakthroughs in the NIH Intramural Research Program.
References:
[1] Resting state connectivity best predicts alcohol use severity in moderate to heavy alcohol users. Fede SJ, Grodin EN, Dean SF, Diazgranados N, Momenan R. Neuroimage Clin. 2019 Mar 19;22:101782. doi: 10.1016/j.nicl.2019.101782. [Epub ahead of print]
Related Blog Posts
This page was last updated on Wednesday, May 24, 2023