Untangling Alzheimer’s Disease — Longitudinal Human Neuroimaging
Alzheimer's disease begins years, even decades, before any symptoms become apparent. And when symptoms begin to manifest, diagnosing the disease is difficult in non-research settings, and a definitive diagnosis can only be made at autopsy. Early detection is therefore paramount to advancing our understanding of Alzheimer’s disease onset and progression, and to enabling the testing of promising interventions.
Advances in neuroimaging technologies now allow researchers to quantify levels of amyloid, and more recently tau, in the living brain. PET (positron emission tomography) imaging of amyloid and tau—the hallmarks of the disease—brings a potentially revolutionary method to quantitatively assess people for early signs of Alzheimer’s. Prior to these PET imaging methods, detailed measurements of the brain amyloid and tau could only be obtained by examining the brain after death.
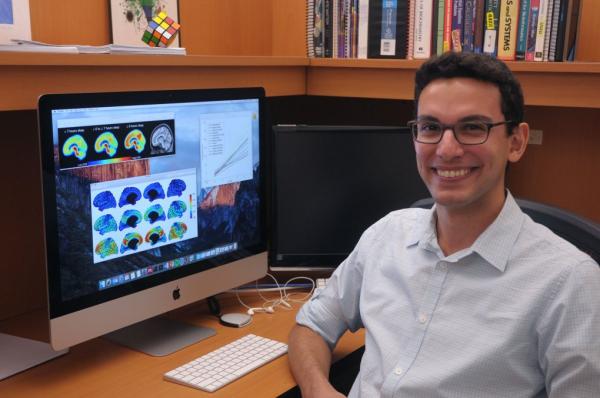
Murat Bilgel, NIH IRP predoctoral fellow, at his desk
“I believe that the Alzheimer’s disease research field is poised to make significant advances in the near future,” says Murat Bilgel, a predoctoral visiting fellow in the IRP’s Laboratory of Behavioral Neuroscience (LBN). “We need sophisticated tools for analyzing the large amounts of neuroimaging and other types of data being collected to make this possible and to accelerate scientific progress. I find this field highly receptive to methodological development, both in terms of statistical modeling and development of neuroimaging analysis techniques.”
Bilgel and colleagues are uniquely positioned to detect the earliest changes in the brains of Alzheimer’s patients. They utilize data obtained as part of the longest running scientific study of human aging in the US: the NIH National Institute on Aging’s Baltimore Longitudinal Study of Aging (BLSA). Researchers follow study participants over time with the goal of understanding biological changes that accompany aging. The availability of extensive data leading up to cognitive impairment and dementia makes the BLSA an indispensable resource for Alzheimer’s research.
“The goal of my project is to study these earliest stages of Alzheimer’s disease by constructing a timeline of changes in brain imaging and cognitive measures related to Alzheimer’s in a group of cognitively normal individuals,” Bilgel explains.
In a recent publication by Bilgel, colleagues, and collaborators, the team developed a statistical model to reveal the temporal trajectories of brain amyloid accumulation. The model helps them to better understand the accumulation of amyloid over time across multiple brain regions, as well as the regional differences in rates of accumulation.
“A temporal biomarker ‘template’ estimate using longitudinal data would allow for a better identification of the disease stage of clinical trial volunteers and patients and potentially enable individualized predictions of disease course,” Bilgel further explains.
The laboratory is currently extending those techniques to other Alzheimer’s-related biomarkers, including structural brain changes, neuronal integrity, tau changes, cognitive measures, and blood and cerebrospinal fluid biomarker measurements. However, a major challenge is that people do not exhibit the same biomarker changes in the same temporal order, and it is difficult to parse out disease states from normal aging processes.
“My future work will be focusing on designing models to capture this heterogeneity so that we can better understand Alzheimer’s-related biomarkers without confounding longitudinal processes,” Bilgel says. “Understanding these earliest changes will enhance our ability to develop ways to prevent and delay Alzheimer’s disease, and may lead to improved therapies.”
References:
Bilgel, M., An, Y., Zhou, Y., Wong, D. F., Prince, J. L., Ferrucci, L., & Resnick, S. M. (2015). Individual estimates of age at detectable amyloid onset for risk factor assessment. Alzheimers Dement, 12(4), 373–379. http://doi.org/10.1016/j.jalz.2015.08.166
Bilgel, M., Prince, J. L., Wong, D. F., Resnick, S. M., & Jedynak, B. M. (2016). A multivariate nonlinear mixed effects model for longitudinal image analysis: Application to amyloid imaging. NeuroImage, 134, 658–670. http://doi.org/10.1016/j.neuroimage.2016.04.001
Related Blog Posts
This page was last updated on Wednesday, July 5, 2023