Crowdsourcing
And Virtual Colonoscopies
When it comes to interpreting the results of virtual colonoscopies, radiologists “have a hard time taking the advice of computer aids,” said senior investigator Ronald Summers, chief of the NIH Clinical Center’s Clinical Image Processing Service. Computer-aided-detection (CAD) technology, which he helped develop for colorectal cancer screening, is supposed to make the radiologist’s job easier. CAD is more effective than humans at finding tiny dings on the scan that represent polyps, but it identifies mock polyps, too—residual fecal matter clinging to the colon wall, thick folds in the colon, or even artifacts such as surgical clips or hip prostheses. Even though radiologists should be able to tell the difference between true and false readings, they often ignore CAD’s true findings.
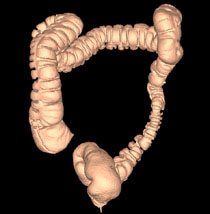
Zhuoshi Wei, CC
Reconstructed CT images create a 3D model of the colon.
Summers wanted to reduce what he suspected were perceptual errors involving the CAD system, but he needed to be able to observe its users in action. Radiologists, however, are too busy to participate in such observer-performance experiments. Then he stumbled on a way to get participants: crowdsourcing, also known as distributed human intelligence. It’s a method for recruiting large numbers of anonymous laypeople, or knowledge workers (KWs), to perform simple tasks distributed over the Internet. Summers reported his findings in a recent issue of Radiology (Radiology 262:824–833, 2012).
“This was one of the most fascinating submissions and papers that we’ve [ever] had,” Radiology editor Herbert Kressel said in a podcast interview with Summers.
Colon cancer, the second leading cause of cancer death in the United States, can be prevented by removing colorectal polyps before they become malignant. Polyps can be detected by either a conventional colonoscopy, in which a colonoscope—a long, flexible, lighted tube with a tiny video camera attached—is inserted into the rectum and guided into the large intestine; or a virtual colonoscopy [computed tomography (CT) colonography], in which a CT scanner creates two- and three-dimensional images of the colon. Both methods require the patient to drink a bowel-clearing preparation the day before to be sure the colon is empty.
After obtaining approval from the Office of Human Subjects Research, Summers developed a project using crowdsourcing to assess how well KWs could correctly identify polyps on CT colonographies. He hired an Internet-based crowdsourcing service, which recruited 228 KWs. The KWs were given just one to two minutes of training to recognize features of polyp candidates in CT colonographic images. The CT data—from 24 randomly selected anonymized patients who had at least one polyp of six millimeters or larger—were analyzed by CAD software, and true polyps were confirmed by conventional colonoscopies.
Summers was surprised that when the results were combined, the minimally trained KWs did about as well as a highly trained CAD system—about 85 percent. (The KWs were only shown 11 candidates for training, the CAD system, more than 2,000.) He even did two trials, four weeks apart, and got the same results.
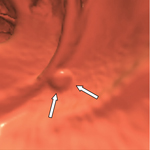
Zhuoshi Wei, CC
The arrows point to a polyp detected in a CT colonography.
Summers will use the insights about how people perceive images to improve CAD systems and develop training programs to help medical personnel more accurately interpret CT colonographic images. He is already doing follow-up crowdsourcing studies to test how well people identify polyps when they can scroll through images as well as see polyp candidates from all angles.
Crowdsourcing studies have “the potential to change how these perception experiments in medical imaging are conducted,” said Summers. “I think the outcomes will be better CAD systems.”
To listen to the podcast of Summers’s interview with Radiology editor Herbert Kressel, visit http://radiology.rsna.org/content/suppl/2012/02/23/radiol.11110938.DC2
This page was last updated on Monday, May 2, 2022