New Methods
Lights, Camera, Mouse Action with SCORHE
NIH Develops New Mouse-Behavior Monitoring System
Automated video-based monitoring of laboratory mouse behavior is getting more efficient thanks to a team of NIH researchers led by Ghadi Salem, a staff scientist in the Signal Processing and Instrumentation Section (SPIS) at NIH’s Center for Information Technology. The new “System for Continuous Observation of Rodents in Home-cage Environment” (SCORHE) is composed of custom video-acquisition and analysis tools that can quantify mice activity and behavior for short and long (multi-day) durations while the mice are housed within a typical home-cage. The specialized hardware was designed to be space efficient, compatible with vivarium cage racks, and animal-facility-user friendly. The advanced software algorithms output animal-behavior measures.
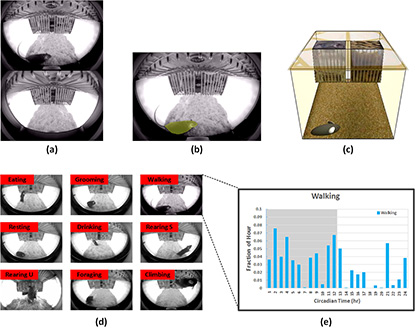
CREDIT: GHADI SALEM, CIT
SCORHE software analyzes incoming video streams to produce detailed time-resolved activity measures for full circadian cycles. (a) A still image from the front-view camera (top) and the rear-view camera (bottom). (b) The first processing module segments the incoming images to identify mouse pixels and determine the view in which the mouse is visible. (c) An advanced estimation algorithm reconstructs the corrected 3D position of the mouse head/tail from the fisheye distorted image. (d) A classifier is trained to inspect a sequence of frames and automatically detect one of nine pre-defined behaviors. (e) Time-resolved profiles are generated for each of the pre-defined behaviors. Shown is the ‘walking’ behavior profile for a 24-hour cycle. The gray shading in the plot indicates the dark cycle, highlighting the nocturnal nature of the mouse.
Video monitoring of animals in their home cages is noninvasive and provides more information about behavior than observations of mice using running wheels or tripping photobeam detectors. SCORHE—which can monitor the day-to-day health of mice, provides advanced behavioral screening, assesses the short- and long-term effects of experimental treatments—avoids disrupting circadian rhythms, does away with the need for mice to become acclimated to test environments, and allows for night-time measurements. Commercially available systems can be expensive and, unlike to SCORHE, do not integrate with vivarium cage-racks.
“SCORHE was designed to demonstrate the feasibility of integrating large-scale, video-surveillance methods in animal facilities by means of efficient mechanical design leveraging a nontraditional camera configuration,” Salem explained in the group’s publication in Behavioral Research Methods (Behav Res Methods 47:235–250, 2015).
“This [system] eliminates issues with moving the mice from their home cage to the testing environment, which can be stressful for them,” said principal investigator Alexxai Kravitz (National Institute of Diabetes and Digestive and Kidney Diseases), who is validating SCORHE’s ability to analyze obesity-related behaviors such as locomotion and feeding.
Lights: To obtain both daytime and night-time measures of behavior, SCORHE uses near-infrared illumination, which provides consistent image quality without disturbing the animals’ light and dark cycles.
Camera: The SCORHE units employ inexpensive Raspberry PI digital cameras fitted with fisheye lenses. Although the fisheye lenses cause images to be distorted, they are key to the compact design and ventilated-rack integration. SPIS engineers developed software for continuous high-frame-rate capture of digital video from multiple cameras over long durations. The software comes with a Python-based Graphical User Interface (GUI) which allows the user to preview video streamed from the cages and set up multi-cage experiments.
Action: The most advanced feature of SCORHE is its ability to extract measures of mouse activity and behaviors. The SCORHE team has developed algorithms to accurately estimate the 3D position of the mouse despite the fisheye-lens distortion. The algorithms also measure bouts of predefined behaviors including walking, grooming, food-interaction, drinking, climbing, and resting. The measures provide detailed time-resolved locomotion and behavior profiles for the mouse over multiple circadian cycles.
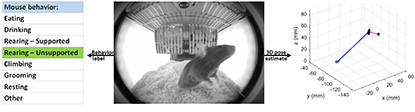
CREDIT: GHADI SALEM, CIT
SCORHE video (center) is analyzed automatically to produce a per-frame 3D-pose estimate (right) and behavior label (left). The pose estimates (estimates of the mouse’s position) and behavior labels are used to generate full circadian-cycle profiles of activity and behavior.
The system can be used for phenotypic characterization of animal models, behavioral and activity profiling, circadian and sleep-pattern monitoring, and therapeutic trials. “Pharmaceutical, biotech, and device companies as well as academic and government researchers want to maximize acquisition of reliable data with every experiment,” said SCORHE team member John Dennis, who is the director of the Division of Veterinary Services at the FDA Center for Biologics and Evaluation Research (Silver Spring, Maryland). “Repeating animal studies costs time, money, and animal lives, but SCORHE provides a way to refine and reduce animal use in a practical and economical way,” he said. “Since behavior is an expression of overall health, our system can demonstrate improvements in health and quality of life and it can make animal studies more humane by identifying and reporting humane endpoints automatically.”
As work is underway to produce the latest set of prototypes, the SCORHE team is preparing for NIH intramural researchers to collaborate on beta testing (on their specific application) to produce a more refined and capable system. The development will be kept open-source to widely serve the NIH intramural program and the greater scientific community. More information can be found at the project website: https://scorhe.nih.gov.
Other papers:
Salem G. et al. (2016). Scalable Vision System for Mouse Homecage Ethology. In International Conference on Advanced Concepts for Intelligent Vision Systems (pp. 626–637). Springer. (https://link.springer.com/chapter/10.1007/978-3-319-48680-2_55)
Salem G. et al. (2016). Three dimensional pose estimation of mouse from monocular images in compact systems. In Pattern Recognition (ICPR), 2016 23rd International Conference on (pp. 1750–1755). (http://ieeexplore.ieee.org/abstract/document/7899889/)
Salem G. et al. (2016). Cascaded regression for 3D pose estimation for mouse in fisheye lens distorted monocular images. In Signal and Information Processing (GlobalSIP), 2016 IEEE Global Conference on (pp. 1032–1036). (http://ieeexplore.ieee.org/abstract/document/7905998/)
This page was last updated on Monday, April 11, 2022