Homegrown AI
NIH Researchers Are Creating AI Tools Benefiting the Broader Biomedical Community
BY THE NIH CATALYST STAFF
Artificial intelligence (AI) is reshaping research across the NIH, with scientists creating tailored tools to meet the demands of their own complex datasets and questions.
Tapping into the power of neural networks, large language models (LLMs), and other AI architectures, NIH researchers are teasing apart single-cell gene expression patterns and denoising fragile microscopy images, to name just a few emerging AI applications.
These tools don’t simply automate what was previously manual; they enable entirely new modes of analysis previously impossible. And in many cases, these homegrown AI solutions—created to solve a local problem—are proving to be useful for the broader biomedical research community.
Such ideas will be on display at the NIH Artificial Intelligence Symposium 2025 on May 16 at Masur Auditorium and on the FAES Terrace. This full-day event will explore a broad range of AI approaches in biomedical science and will feature two external keynote speakers and a smattering of intramural research posters.
Read on for a sample of four homegrown AI tools among the dozens pouring out of today’s NIH labs.
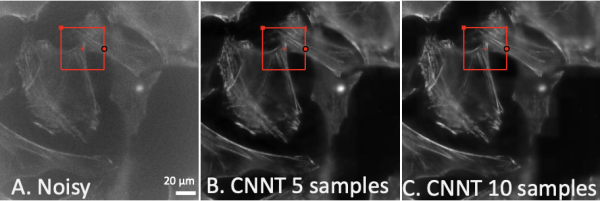
CREDIT: SCIENTIFIC REPORTS
Coming into focus. Seen here are mouse embryonic fibroblast cells in increasingly clear resolution. The first (A) is a low-quality noisy image as an input for the model. Images B and C show the improved clarity from the convolutional neural network transformer after it was trained for 30 cycles using five and ten training images, respectively.
I can see clearly now, the noise is gone
Scientists and engineers have made incredible strides in microscopy, with biological imaging close to the molecular level. They have probed not only more deeply but also more gently, with techniques such as fluorescent labeling and light-sheet microscopy that impart less photon damage to the objects imaged, enabling the study of live cells in unprecedented detail.
Nevertheless, fluorescent signals can restrict both spatial and temporal resolution, and researchers often rely on low light levels during imaging to minimize photobleaching and reduce cellular stress, which is critical when working with live, moving samples. This results in “noisy” or fuzzy images.
One common AI technique to denoise microcopy images, called convolutional neural networks (CNNs), a type of deep learning model, has had limited effectiveness because it can be time-consuming, tedious, and, in the end, rather image-specific. Hoping to develop a tool that would be faster and more broadly applicable, researchers at the NIH and their external colleagues created what has become known as convolutional neural-network transformer (CNNT). As the name implies, this deep-learning model added a “transformer” tool to the convolutional neural networks. The transformer tool has been famously used in ChatGPT to help computers understand language, such as figuring out what a sentence means or predicting the next word. But the tool also is ideal to help decode complex patterns in images.
With the CNNT, this team—comprising researchers at NHLBI, NIBIB, FDA, HHMI, Janelia, and Microsoft—devised a denoising system based on a single, strong “backbone” model using many examples from one microscope. From this backbone—analogous to a human skill, such as piano playing, which can be adapted to play classical or jazz or pop—other microscopes can be trained with just a few new images.
“This saves enormous effort in training time and resources for each new experiment,” said Chris Combs, director of the NHLBI Light Microscopy Core, who co-led the development of the tool.
The team demonstrated CNNT’s performance on three types of microscopy: Wide-field imaging of mouse cells, two-photon imaging of zebrafish (Danio rerio) embryos, and confocal imaging of mouse lung tissue. Perhaps the most exciting application lies in large-scale imaging tasks. In one experiment, CNNT enabled a sevenfold increase in imaging speed while maintaining image fidelity, a breakthrough for working with fragile live samples or time-lapse recordings.
A paper describing the CNNT tool was published in August 2024 in Scientific Reports (PMID: 39107416) and features, among many, first author Azaan Rehman, a former postbac with NHLBI, and senior author Hui Xu, former director of the NHLBI AI Core, now at Microsoft.
Combs said his team hopes to improve their CNNT model by scaling it up with larger and more diverse training datasets, which could eventually lead to a light microscopy foundation model with broader applicability.
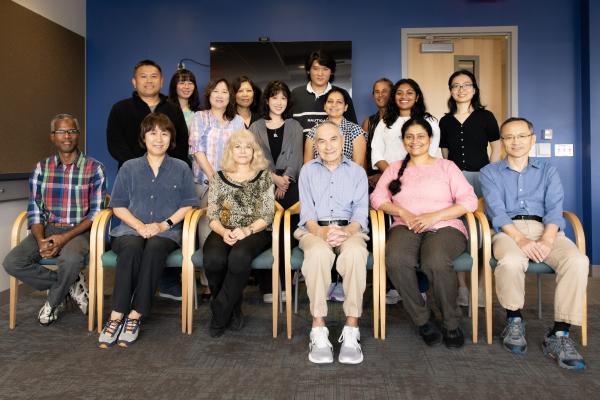
CREDIT: DUSTIN HAYS, NEI
This group photo features members of Rachel Caspi’s (front row, center) lab, including Vijay Nagarajan (front row, far left) and Guangpu Shi (front row, far right).
Help is on the way for single-cell analysis
Members of NEI Laboratory of Immunology, led by Rachel Caspi, have developed a tool called SCassist, an AI-powered workflow assistant for single-cell analysis. Its first application has been to probe the immune function of Müller cells in the retina, the results of which will be published in the coming months. But the tool itself will be broadly useful to anyone drowning in single-cell RNA sequencing (scRNA-seq) data, the team said.
First, the science. Müller cells are the principal glial cells in the retina. They help to maintain retinal structure and physiological homeostasis and support neuronal function. During retinal injury or disease, these cells can shift to a state called reactive gliosis and perform immune-like functions such as inflammatory signaling. Their varying activation states coupled with the unique retinal environment—comprising disparate layers of retinal cells, a blood–retina barrier, and numerous immune cells interacting in space and time—make it difficult to discern their precise role in immunity.
Caspi and her collaborators had discovered that Müller cells can profoundly inhibit inflammatory lymphocytes, capable of attacking the retina, through cell–cell contact. However, the inhibitory molecules remained unidentified, largely because the technology to reveal them in a comprehensive fashion had not yet been developed.
To tackle this problem, Caspi’s group turned to scRNA-seq to determine gene expression profiles of individual Müller cells, initially by re-analyzing a publicly available scRNA‑Seq dataset of Müller cells from eyes with autoimmune inflammation. This analysis could reveal the potential of these cells to express immune-related genes and functions and help demonstrate how Müller cells use them to inhibit inflammatory lymphocytes.
The problem with scRNA-seq, however, is that the investigators are blessed with an abundance of data—thousands of genes in thousands of cells—that is difficult to analyze. Caspi’s team started analyzing the scRNA-seq data several years ago using the standard tools available, which was cumbersome and laborious and not ideal to make system-level interpretations.
With the rise of AI, Vijay Nagarajan, a staff scientist in Caspi’s lab specializing in bioinformatics, had an idea: apply the power of LLMs to create an assistant that would help analyze the complex scRNA-seq data and provide humanlike interpretations, connecting dots.
The AI tool that Nagarajan created and has now patented, SCassist, is built on Google’s Gemini and Meta’s Llama3. He worked with fellow NEI staff scientist Guangpu Shi, who had spent years studying ocular cells involved in immune responses. Shi used SCassist to create a comparison matrix for a detailed functional analysis of Müller cell subpopulations.
Among their findings, the team identified nine distinct Müller cell subgroups; found that three of them are activated in an inflamed retina; found two distinct activated Müller cell phenotypes with macrophage-like properties, governed by Neurod1 and Irf family transcription factors; and learned about key interactions with helper T lymphocytes.
“The ability to combine the power of scRNA-seq with AI is a complete game changer,” Caspi said.
Nagarajan and Shi have since teamed up to create IAN, which they describe as an “R package designed to perform contextually integrated ’omics data analysis and reasoning,” building on SCAssist and leveraging the power of LLMs to unravel complex biological systems.
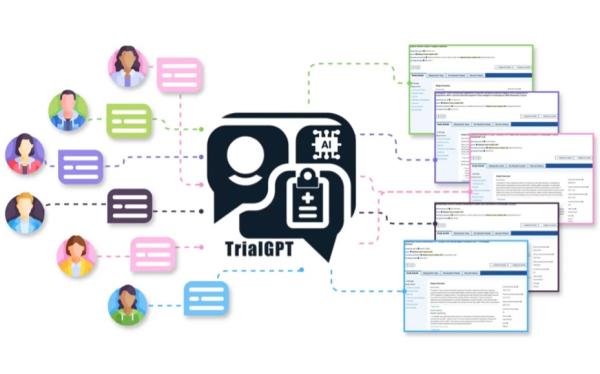
CREDIT: ZHIYONG LU
Are you being served?
Zhiyong Lu, a senior investigator who leads the NLM Biomedical Text Mining Research Group, has been creating AI tools since his arrival at the NIH in 2007. The “Best Match” button for PubMed searches is one of the tools his team created. This tool, introduced in a 2018 PLoS Biology paper (PMID: 30153250), uses natural language processing (NLP) and other AI-based algorithms to better understand the intent behind a user’s search query and match it with relevant articles.
But Lu says much bigger plans are on the horizon. “In my 20 years of research with AI and NLP, we have never seen any AI algorithm so powerful and so amazing [than what’s out now] in terms of its capability for text understanding and generation. Period.”
Tapping into this power of recent LLMs such as ChatGPT, Lu’s group created TrialGPT to streamline the process of matching patients to clinical trials. There have been attempts to accomplish this important and seemingly straightforward task using AI, but none have resulted in an accurate product that warrants its use in practical applications.
Qiao Jin, a postdoctoral fellow in Lu’s group, approached the task by having the AI tool analyze patient notes against each single inclusion and exclusion criterion of a trial protocol and then forcing AI to not only determine who would be eligible for a match—that is, yes or no—but also state why the decision was made for improved explainability and transparency. The “asking why” part prompted the AI tool to better assess its output, resulting in a matching accuracy of more than 80%, comparable to human experts, but in a fraction of the time.
The November 2024 Nature Communications paper (PMID: 39557832) describing this tool was featured in the journal’s “Editors’ Highlights” and ranked among the top 25 most downloaded health science papers last year. See the NIH Director’s Blog from last December for a rewarding summary.
Lu said this unique tool will assist, not replace, doctors and nurses by saving time and making patient connections that otherwise could have been missed.
Another AI tool that Lu’s group is working on—and they have many, in collaborations across the NIH and beyond—is for gene-set analysis, a routine task for interpreting high-throughput data to understand the collective function for a set of genes, not just one. The go-to method has been a statistical enrichment analysis based on databases of known gene-set functions. However, when the database does not contain a significant subset of the genes in question, the statistical analysis is on shaky ground.
Standard LLMs can already predict function as effectively as the aforementioned statistical analysis rather quickly and even explain its reasoning. “While impressive, these results are not always reliable, as they remain vulnerable to hallucinations,” Lu said. That is, even the “why” could be a fabricated answer.
In response, Zhizheng Wang, another postdoctoral fellow in Lu’s group, proposed GeneAgent, an LLM-based tool featuring a built-in AI agent that automatically retrieves relevant gene-centric knowledge from expert-curated databases to verify initial predictions and make corrections when necessary. By doing so, GeneAgent effectively minimizes hallucinations and enhances performance. Lu’s group has a preprint describing GeneAgent (PMID: 38903746), which is expected to be published in Nature Methods.
Lu’s group addresses the challenges of implementing LLMs in health care in an April article in the journal Annual Review of Biomedical Data Science (PMID: 40198845). Said Lu, simply, “I want our AI tools to be used.”
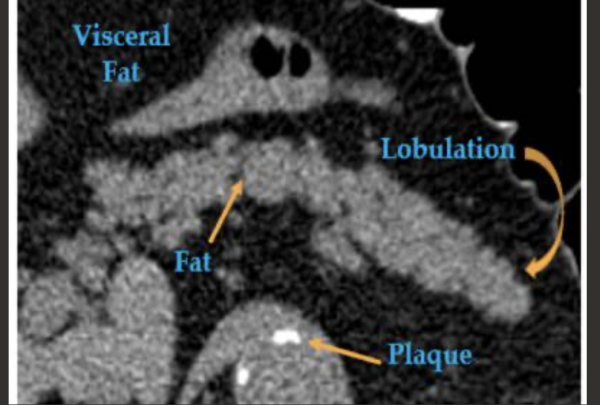
CREDIT: RADIOLOGY
Pancreatic computed tomography scans can help predict type 2 diabetes: Images in a 67-year-old man with type 2 diabetes who was diagnosed 595 days after CT. This pancreas is relatively inhomogeneous due to fatty atrophy, lobulated, and irregular in contour.
Predicting diabetes on CT scans
The letters “CT” are not in “diabetes,” and you may be surprised to learn of a connection. A team of NIH Clinical Center researchers and their colleagues have used AI to predict type 2 diabetes in patient computed tomography (CT) scans with an accuracy of upward of 90%.
While there are other more accurate ways to diagnose diabetes, the novelty of this method is that it reveals the promise of predicting chronic disease from CT scans originally performed for unrelated reasons. Also, diabetes is a disease often diagnosed late, when organ damage has already begun, but this AI approach can reveal biomarkers from abdominal CT scans that are strongly predictive of disease status even years in advance.
The work, led by Ronald Summers, a senior investigator in the Clinical Center’s Imaging Biomarkers and Computer-Aided Diagnosis Laboratory, in collaboration with Perry Pickhardt at the University of Wisconsin at Madison, builds upon incremental advances first in automating the detection of CT biomarkers in and around the pancreas that point to type 2 diabetes (PMID: 35380492), then developing AI models to trace the pancreas in CT images, a task called segmentation, which is complicated by the pancreas’ small and irregular, wrinkled shape (PMID: 38944630).
For this latter part, Summers and his team tested five segmentation models on more than 350 scans from a database of Clinical Center patients. Three models—TotalSegmentator, AAUNet, and AASwin—consistently performed the best, producing pancreas outlines that closely matched those drawn by radiologists. With very accurate outlines, the AI tool could measure fat, tissue density, and the presence of nodules on the organ surface, all potential indicators of diabetes.
With models in hand, the team put the method to the test in a retrospective study of more than 9,700 patients at two medical centers, the Clinical Center and the University of Wisconsin School of Medicine and Public Health. They compared biomarkers derived from three segmentation algorithms and trained machine learning models to predict whether patients had diabetes at the time of the scan or developed it within four years.
Despite variations in segmentation, the key attenuation-based biomarkers—particularly average pancreas density and intrapancreatic fat fraction—showed high agreement across models and a strong predictive performance, with positive predictive values up to 84% and negative predictive values as high as 94%.
There is no standard clinical practice for visually diagnosing or predicting diabetes from pancreas morphology on CT. Therefore, this AI approach isn’t outperforming radiologists per se; it’s enabling a type of analysis that would otherwise be impractical or unavailable in routine clinical care. Manual segmentation of the pancreas is extremely labor-intensive and rarely done outside of research.
The findings, which will appear in Academic Radiology (PMID: 40121118), underscore the potential for using routine CT scans opportunistically to screen for chronic disease. The approach could be integrated into clinical workflows without needing scan protocols tailored to diabetes detection.
This team—which includes Pritam Mukherjee, an associate scientist in Summers’ lab, and now extramural collaborators in NIDDK’s T1DAPC/DREAM study and in the Consortium for the Study of Chronic Pancreatitis, Diabetes, and Pancreatic Cancer (PMID: 30325859)—are now investigating the use of their algorithms for patients with acute or chronic pancreatitis.
Summers noted that as segmentation algorithms become more accurate and generalizable, CT-based biomarker extraction could be applied to detect or predict a wide range of conditions—from metabolic syndromes to malignancies—all without additional patient burden. These studies mark a key advance in using AI to transform existing radiological data into predictive tools for preventive medicine.
NIH Artificial Intelligence Symposium 2025
Interested in how AI can be applied to your research? Ideas will be flowing at the NIH Artificial Intelligence Symposium 2025 on Friday, May 16, at Masur Auditorium and on the FAES Terrace. The full-day event will feature two external keynote speakers: Alexander Rives, cofounder and chief scientist at Evolutionary Scale, a company focused on applying machine learning and language models to biological systems; and Leo Anthony Celi, clinical research director at the MIT Laboratory for Computational Physiology (Cambridge, Massachusetts)and associate professor of medicine at Harvard Medical School (Boston), whose interests include integrating clinical expertise with data science.
Learn more by downloading the NIH Artificial Intelligence Symposium 2025 Program.
This page was last updated on Sunday, May 18, 2025