Algorithms for Life
Teresa Przytycka answers complex biological questions using computational tools.
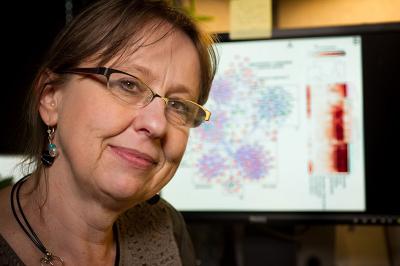
Senior Investigator Teresa Przytycka, Ph.D.
Throughout centuries of biomedical discovery, investigators have looked to the physical sciences for new tools to pull their fields forward. When biologists needed to see cells in more detail, physicists designed better lens and laser technologies. When they started to think about molecular manipulation, chemists created tools for cleaving DNA. As the “-omics” revolution ushers in enormous amounts of new biological data, computer scientists provide methods of analysis, storage, and applied access to the terabytes of genomic, proteomic, and metabolomic information.
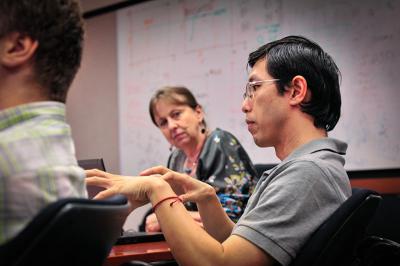
Yang Huang, Ph.D., discusses computational biology with Dr. Przytycka
Several years ago, while visiting the Center for Discrete Mathematics and Theoretical Computer Science, Teresa Przytycka, Ph.D., a computational scientist with a background in advanced theoretical algorithms, was approached with a question related to evolutionary biology. “Have you heard of evolutionary trees?” the researcher asked. At the time, Przytycka was working on new algorithms involving a data structure that computer scientists refer to as a tree. “I told him I’d never really thought about it, but that I could see how his question related to my research,” Przytycka says. And with that innocuous question, she began her second career as a computational biologist.
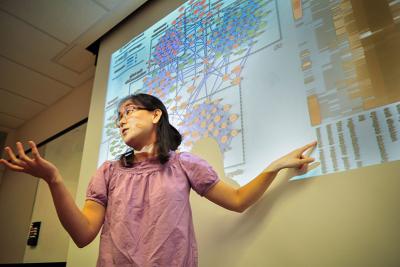
Postdoctoral Fellow Yoo-Ah Kim, Ph.D., presents research findings
Evolutionary biology was the first biological field to really embrace computational biology. When the basis of inferring evolutionary trees changed from phenotype (the outward appearance and traits of an organism) to genotype (the genetic components that determine the phenotype), the need for computational analysis became clear. DNA sequences—the vast strings of the four component nucleotide bases: adenine (A), guanine (G), cytosine (C), and thymine (T)—are defined inputs that lend themselves to computational analysis. It quickly became standard to take genomic data and use it to estimate evolutionary distances. Computational algorithms to infer and compare evolutionary trees followed.
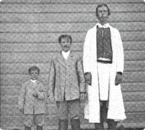
How do genotypic variations result in different phenotypes, such as the genetic basis for height?
“Of course, this revolution in biology was also an evolution in computational biology,” explains Przytycka. “Computational biology couldn’t evolve without data, and the well-defined biological data of the genome was crucial in allowing computational biologists access to this new world.” Since then, computational biologists have continued to provide insight in many fields of biology, as new data becomes available. “Computational biologists are, in a sense, a little opportunistic—what you can contribute depends on what data is available to you,” notes Przytycka. At the rate that IRP scientists produce data, opportunities for collaboration tend to pile up on Przytycka’s desk.
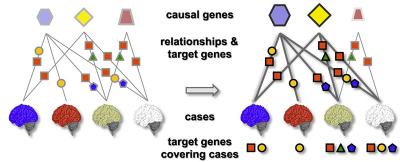
Dr. Przytycka’s team developed a novel computational method to identify causal genes and associated dysregulated pathways
Przytycka and her colleagues apply their knowledge to many different fields of biology, but most commonly in an area of research known as systems biology. In systems biology, computational biologists work to understand how changes at one level can affect the system as a whole, for instance, how a single perturbation—in DNA, RNA, or protein—permeates the many pathways of the biological system to result in an altered phenotype (physical or behavioral trait, disease symptom, tumor). Computational biologists investigate perturbations at multiple levels: molecular, system, associations, populations, and, eventually, evolution.
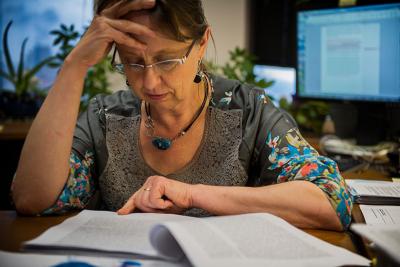
Dr. Przytycka surveys recent literature
The real-world application of computational biology is immensely powerful. Recently, Przytycka investigated how the same disease might develop via very different genetic perturbations. “In this work, we used a systems level approach to identify causal genes, based on knowing which pathways are disrupted within individual tumors,” Przytycka says. Using a bank of 128 glioma tumors, she and her team identified genes that were differentially expressed in the tumor samples compared to controls. They then used a series of complex algorithms based on electrical circuits to identify candidate causal genes and pathways that may be responsible for the altered expression of disease genes in glioma. Challenging the current paradigm of looking for similarities in tumors, her group also focuses on the heterogeneity of the disease, modeling each disease case as a mixture of disease subtypes.
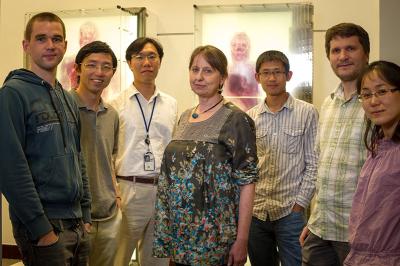
The team: Jan Hoinka, Yang Huang, Dong-Yeon Cho, Teresa Przytycka, Xiangjun Du, Damian Wojtowicz, Yoo-Ah Kim (not pictured, Phuong Dao)
Not only has Przytycka’s research demonstrated a novel approach to identifying causal disease genes and dysregulated pathways, it also placed the complex algorithmic software in the public domain. “Anyone will be able to access this algorithm, and any others we create, and use it in their own research,” Przytycka says. “At the end of the day, we’re a computational group in a sea of experimentalists, and if we can help others by making our tools freely available then we’re delighted to be able to do that—that’s pretty much what the IRP is all about, isn’t it?”
Teresa M. Przytycka, Ph.D., is a Senior Investigator and Head of the Algorithmic Methods in Computational and Systems Biology Section in the Computational Biology Branch of the National Center for Biotechnology Information (NCBI) at the National Library of Medicine (NLM).
This page was last updated on Wednesday, May 24, 2023