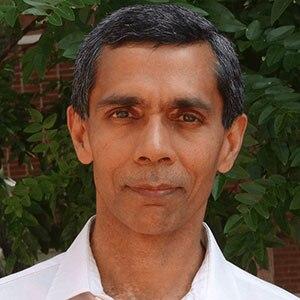
Vipul Periwal, Ph.D.
Senior Investigator
Computational Medicine Section, Laboratory of Biological Modeling
NIDDK
Research Topics
The ultimate goal of our research is to predict quantitatively the effects of therapeutic interventions in human disease.
Current Research
Data-driven large-scale biological modeling of disease
Biology is complex. For example, diabetes is the end result of a long process of increasing insulin resistance culminating in an inability to produce sufficient insulin to keep glucose levels under control. This increasing insulin resistance is the result of several interacting processes that are still being sorted out, such as increasing fat mass and increasing mitochondrial dysfunction. At the cellular level, at the organ level and at the whole body level, predictive models of this dysfunction are important to integrate different scales in space and time into a coherent whole. New technologies provide increasingly detailed data on underlying biological signaling networks. Extracting predictive models of disease causes from such large-scale data directly is a difficult but essential problem in modern biology. The goal of our research is to deduce data-driven unbiased mathematical models that can be used to help find interventions that can guide therapy for complex diseases.
Model of Reactive Oxygen Species in Mitochondria
Reactive oxygen species (ROS) have been shown to have tissue-damaging effects that underlie many disease complications, including those associated with diabetes, Parkinson's, Alzheimer's, and atherosclerosis (Brownlee, 2005). This oxidative stress is thought to result from an organism's inability to detoxify and repair damage at the same rate that ROS are produced. On the other hand, it should be noted that ROS signaling is important in cellular functioning. In mitochondria, where ROS (e.g., superoxide) are produced through a process that is very sensitive to the proton motive force, oxidative stress is prevented by scavenging enzymes (e.g., MnSOD) and uncoupling proteins (e.g., UCP2). Details of this regulation in mitochondria are still being established. The interplay between nutrient sensing and ROS signaling is complex, and the goal of our research is to mathematically model the relevant pathways to understand the deleterious aspects of this interplay as it relates to the metabolic syndrome and obesity.
Adipocyte Development and Insulin Resistance
Our overall goal is to understand how adipose tissue dynamics are related to insulin resistance and diabetes. Adipose tissue grows by two mechanisms: hyperplasia (cell number increase) and hypertrophy (cell size increase). How do genetics and diet affect the relative contributions of these two mechanisms to the growth of adipose tissue in obesity? We are particularly interested in investigating the role played by insulin-sensitizing agents such as thiazoledinediones in altering the development of adipocytes. We chose to investigate this dynamic behavior by mathematically modeling the changes in cell size distributions in adipose tissue over time under several conditions, since this will provide a global view of cell size dynamics as adipocytes accumulate lipids and move from small sizes to maturity.
Biography
- Assistant Professor, Physics Department, Princeton University, 1993-2001
- Member, The Institute for Advanced Study, 1991-1993
- Research Physicist, Institute for Theoretical Physics, University of California, 1988-1991
- Ph.D., Princeton University, 1988
- M.A., Princeton University, 1984
- B.S., California Institute of Technology, 1983
Selected Publications
- Aggarwal M, Periwal V. Tight basis cycle representatives for persistent homology of large biological data sets. (external link) PLoS Comput Biol. 2023;19(5):e1010341.
- Jo J, Wagemakers A, Periwal V. Annealing approach to root finding. (external link) Phys Rev E. 2024;110(2-2):025305.
- Aggarwal M, Striegel DA, Hara M, Periwal V. Geometric and topological characterization of the cytoarchitecture of islets of Langerhans. (external link) PLoS Comput Biol. 2023;19(11):e1011617.
- Aggarwal M, Periwal V. Dory: Computation of persistence diagrams up to dimension two for Vietoris-Rips filtrations of large data sets. (external link) J Comput Sci. 2024;79.
- Ali RO, Quinn GM, Umarova R, Haddad JA, Zhang GY, Townsend EC, Scheuing L, Hill KL, Gewirtz M, Rampertaap S, Rosenzweig SD, Remaley AT, Han JM, Periwal V, Cai H, Walter PJ, Koh C, Levy EB, Kleiner DE, Etzion O, Heller T. Longitudinal multi-omics analyses of the gut-liver axis reveals metabolic dysregulation in hepatitis C infection and cirrhosis. (external link) Nat Microbiol. 2023;8(1):12-27.
Related Scientific Focus Areas
Biomedical Engineering and Biophysics
View additional Principal Investigators in Biomedical Engineering and Biophysics
This page was last updated on Monday, April 21, 2025