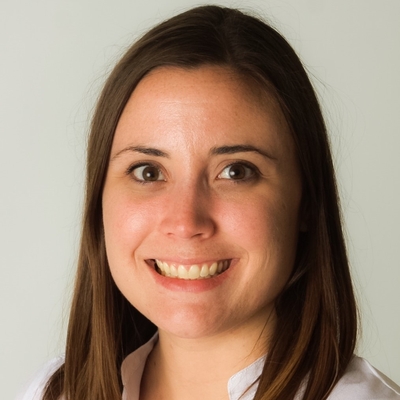
Research Topics
The increasing ability to acquire and rapidly analyze digital pathology images has enabled development of robust machine learning algorithms for automated image analysis. These machine learning tools can aid clinicians and researchers in assessing primary diagnosis and cancer-related outcomes. There is strong evidence that histology features can also be used as surrogate measures for tumor biology. Our long term goal is to identify interpretable imaging-based biomarkers on histopathological images that can predict underlying molecular phenotypes and translate these findings to improve diagnosis, treatment, and patient outcomes in various cancers.
A few areas we will apply these techniques include:
- Integration of digital pathology and molecular phenotypes in prostate cancer. After patients are treated for localized prostate cancer, a subset of them will go on to develop distant metastasis and can undergo various treatments depending on their prior treatment history and aggressiveness of their cancer. As resistance to multiple lines of treatment develops, the patho-biological heterogeneity across patients increases. We aim to identify important histological features of prostate cancer by interrogating its relationship with underlying biological phenotypes derived from molecular assays at various stages of disease. We aim to use these findings to provide useful information to clinicians on early detection of aggressive phenotypes, biopsy targeting, histological interpretation, and treatment selection.
- Evaluating morphological characteristics of aggressive bladder cancer. Bladder cancer is often diagnosed on transurethral resection tissue in early-stage cancers. This information is used by the physician to guide decision making on future surgery and chemotherapy needs of the patient. We aim to integrate digital pathology assessment with clinical and radiographic appearances to better diagnose cancer and inform treatment decisions.
- Explore validation of digital pathology biomarkers using spatial biology platforms. Typical histological assessment is completed on Hematoxylin and Eosin (H&E) images. By spatial profiling the biology of disease through various protein-based and molecular-based assays, we aim to apply our spatial analysis techniques broadly to new imaging platforms. This work will help to validate the biological underpinnings of H&E machine learning models and also help the research community develop tools which can bridge the analysis gap between anatomical and biological findings.
Biography
Dr. Harmon obtained her Ph.D. in medical physics at the University of Wisconsin – Madison in 2016. For her postgraduate work, Dr. Harmon joined Leidos Biomedical Research where she worked within the Molecular Imaging Branch and investigated computational biomarkers in various state-of-the-art prostate imaging modalities. She received many awards during her training, including the Prostate Cancer Foundation Young Investigator Award and the NCI Director’s Intramural Innovation Award. In 2020, she became a staff scientist within the newly formed Artificial Intelligence Resource of NCI. This group excels in development and translation of imaging methods in cancer, supporting intramural investigators in development of AI-based solutions.
Selected Publications
- Patel P, Harmon S, Iseman R, Ludkowski O, Auman H, Hawley S, Newcomb LF, Lin DW, Nelson PS, Feng Z, Boyer HD, Tretiakova MS, True LD, Vakar-Lopez F, Carroll PR, Cooperberg MR, Chan E, Simko J, Fazli L, Gleave M, Hurtado-Coll A, Thompson IM, Troyer D, McKenney JK, Wei W, Choyke PL, Bratslavsky G, Turkbey B, Siemens DR, Squire J, Peng YP, Brooks JD, Jamaspishvili T. Artificial Intelligence-Based PTEN Loss Assessment as an Early Predictor of Prostate Cancer Metastasis After Surgery: A Multicenter Retrospective Study. Mod Pathol. 2023;36(10):100241.
- Harmon SA, Gesztes W, Young D, Mehralivand S, McKinney Y, Sanford T, Sackett J, Cullen J, Rosner IL, Srivastava S, Merino MJ, Wood BJ, Pinto PA, Choyke PL, Dobi A, Sesterhenn IA, Turkbey B. Prognostic Features of Biochemical Recurrence of Prostate Cancer Following Radical Prostatectomy Based on Multiparametric MRI and Immunohistochemistry Analysis of MRI-guided Biopsy Specimens. Radiology. 2021;299(3):613-623.
- Sarma KV, Harmon S, Sanford T, Roth HR, Xu Z, Tetreault J, Xu D, Flores MG, Raman AG, Kulkarni R, Wood BJ, Choyke PL, Priester AM, Marks LS, Raman SS, Enzmann D, Turkbey B, Speier W, Arnold CW. Federated learning improves site performance in multicenter deep learning without data sharing. J Am Med Inform Assoc. 2021;28(6):1259-1264.
- Harmon SA, Sanford TH, Brown GT, Yang C, Mehralivand S, Jacob JM, Valera VA, Shih JH, Agarwal PK, Choyke PL, Turkbey B. Multiresolution Application of Artificial Intelligence in Digital Pathology for Prediction of Positive Lymph Nodes From Primary Tumors in Bladder Cancer. JCO Clin Cancer Inform. 2020;4:367-382.
- Harmon SA, Brown GT, Sanford T, Mehralivand S, Shih JH, Xu S, Merino MJ, Choyke PL, Pinto PA, Wood BJ, McKenney JK, Turkbey B. Spatial density and diversity of architectural histology in prostate cancer: influence on diffusion weighted magnetic resonance imaging. Quant Imaging Med Surg. 2020;10(2):326-339.
Related Scientific Focus Areas
Biomedical Engineering and Biophysics
View additional Principal Investigators in Biomedical Engineering and Biophysics
This page was last updated on Thursday, October 12, 2023