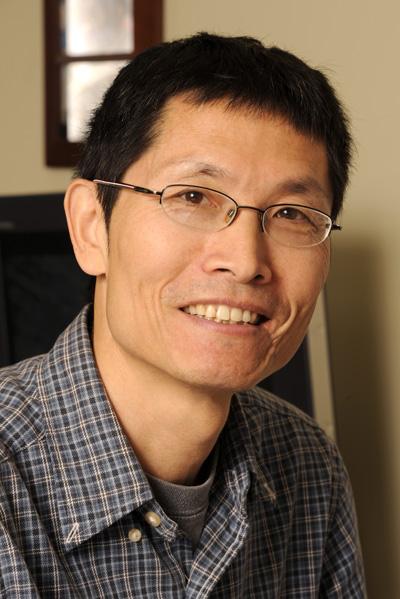
Research Topics
My main focus has been on methods for identifying transcription factor binding sites in sequences. This area offers an interesting and challenging set of methodological problems as well as the opportunity to work closely with laboratory scientists who are developing an understanding of mechanisms of transcriptional regulation. Transcription factor binding sites are short functional elements (5-30 base pairs) in the genomes which, when bound by proteins, induce or repress gene transcription. Transcription controls temporal and spatial gene expression; thus, it is vital to many cellular processes. We have been working on methods to improve the accuracy of transcription factor binding site identification. Specifically, we developed an efficient algorithm for identifying conserved segments between two promoter sequences. Like many others, we reasoned that functional elements are more likely to be found in conserved regions than non-conserved regions. Similarly, we worked on methods that improve the quality of statistical models for binding site identification. We developed a publically available tool for de novo discovery of transcription factor binding sites in a set of DNA sequences based on statistical over-representation of the binding sites without requiring prior knowledge on where the binding sites are located and what they look like. Recently, we developed a statistical method for identifying transcription co-regulators' motifs in ChIP-seq data. It is known that multiple transcription factors may work together to regulate gene expression in development and specification. Most existing methods for motif discovery consider only one motif at a time. We have developed a multi-component mixture framework to model the joint distribution of two motifs. We classify a sequence as containing either motif 1 or motif 2, both motifs 1 and 2, or pure statistical "noise".
We are also developing methods and tools for the analysis of next-generation sequencing (NGS) data, in particular, statistical/computational methods that detect differential changes not only in gene expression but also in splicing patterns from mRNA-seq data. Tools for detecting differential splicing could have a major impact in toxicogenomics, as examples exist where changes or imbalances in isoforms have been implicated in tumor development.
We also have long-standing interests in mining high dimensional genomic data, pattern recognition and classification. Recently, we started a new project that addresses the research question: what is the biological relevance of those CTCF sites that are bound by CTCF (CCCTC-binding factor) in most or all cell lines? In other words, what biological processes/functions does CTCF regulate that are fundamental to most cell lines? Knowing the functional relevance of the constitutive CTCF sites could have far-reaching scientific impact. This potentially would allow us to identify 1) any biological processes that require universal participation of CTCF; 2) other proteins and epigenetic marks that are associated with such processes; 3) the genomic loci at/near where CTCF participates in the processes. Furthermore, the computational methods that we develop in this endeavor will be useful for pooled analyses of hundreds to thousands of ChIP-seq datasets that have been and will be generated.
Biography
Dr. Li obtained his Ph.D. in medicinal chemistry at the University of North Carolina at Chapel Hill. He has a broad background in statistics, biology, computer sciences and chemistry. His research focuses on statistical and computational methods for identifying transcription factor binding sites in ChIP-seq data and for analyzing mRNA-seq data. He has long-standing interests in mining high dimensional genomic data, pattern recognition and classification.
Selected Publications
- Shats I, Williams JG, Liu J, Makarov MV, Wu X, Lih FB, Deterding LJ, Lim C, Xu X, Randall TA, Lee E, Li W, Fan W, Li JL, Sokolsky M, Kabanov AV, Li L, Migaud ME, Locasale JW, Li X. Bacteria Boost Mammalian Host NAD Metabolism by Engaging the Deamidated Biosynthesis Pathway. Cell Metab. 2020;31(3):564-579.e7.
- Kang K, Meng Q, Shats I, Umbach DM, Li M, Li Y, Li X, Li L. CDSeq: A novel complete deconvolution method for dissecting heterogeneous samples using gene expression data. PLoS Comput Biol. 2019;15(12):e1007510.
- Huang W, Li L, Myers JR, Marth GT. ART: a next-generation sequencing read simulator. Bioinformatics. 2012;28(4):593-4.
- Gilchrist DA, Dos Santos G, Fargo DC, Xie B, Gao Y, Li L, Adelman K. Pausing of RNA polymerase II disrupts DNA-specified nucleosome organization to enable precise gene regulation. Cell. 2010;143(4):540-51.
- Li L, Weinberg CR, Darden TA, Pedersen LG. Gene selection for sample classification based on gene expression data: study of sensitivity to choice of parameters of the GA/KNN method. Bioinformatics. 2001;17(12):1131-42.
Related Scientific Focus Areas
This page was last updated on Friday, April 12, 2013