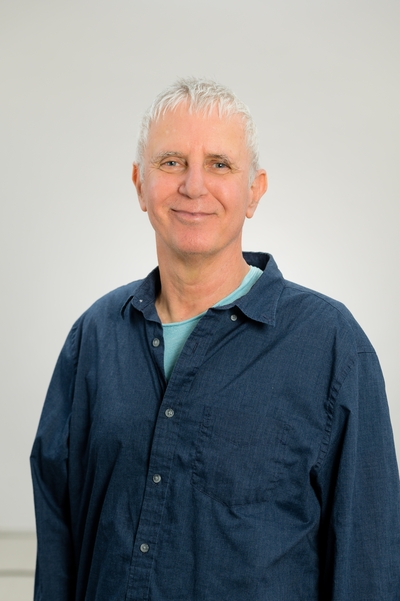
Research Topics
(1) Development and testing new transcriptomics-based precision oncology (PO) approaches: The goal is to broaden the scope of precision oncology (PO) to the realm of tumor transcriptomics and pathology slides.
(1a) Bulk expression-based PO: We developed SELECT and ENLIGHT, aiming to identify synthetic lethality vulnerabilities as a new way for stratifying patients to treatment from the transcriptome of a patient’s tumor. We are in the final approval steps for starting a prospective clinical trial study by the end of the year, focused initially on breast cancer.
(1b) Single-cell based PO: To begin addressing tumor heterogeneity in guiding patient treatment, We developed a new computational approach called PERCEPTION that builds treatment response models based on patients’ single-cell (SC) tumor transcriptomics [https://www.biorxiv.org/content/10.1101/2022.01.11.475728v1]. We show that PERCEPTION successfully stratifies patients to combination therapies and captures the development of clinical resistance based on the patients’ tumor’s SC-expression.
(1c) SC expression-based identification of cancer targets and combination treatments: We developed MadHitter to uncover selective combinations of targets for CAR-T, conjugated antibodies and coated nanoparticle therapies and have further developed this approach to identify new CAR-T targets in head & neck cancer https://www.biorxiv.org/content/10.1101/2021.09.29.462485v2] and currently working with collaborators to test selected predictions.
(1d) Guiding PO from tumor histopathology images: we developed a predictor of cancer patients’ treatment response, termed ENLIGHT-DeepPT, from their H&E slides [https://www.biorxiv.org/content/10.1101/2022.06.07.495219v3]. This new approach could possibly augment the feasibility of precision oncology in developing countries and underserved areas.
(2) The computational study of immunotherapy has two main research aims:
(2a) Transcriptomics-based cell-type specific characterization of the tumor microenvironment (TME): We recently developed CODEFACS, a tool for deconvolving cell type–specific gene expression in each individual tumor sample from its bulk expression, and LIRICS, a statistical framework prioritizing clinically relevant ligand–receptors.
(2b) Transcriptomic Liquid Biopsies (TLB): We are studying what can we learn about the TME transcriptome from matching while blood cell (WBC) expression of patients.
(3) Collaborative multiomics studies of cancer
Together with some of our collaborators, we have previously identified new SL-based treatments for uveal melanoma (the Gutkind lab) and pancreatic cancer (the Ronai lab), which are now in clinical trials. More generally, our collaborative work is devoted to help our experimental and clinical collaborators to extend their lab and animal model findings via a complementary analysis of patients’ data. These collaborations span many labs, both nationally and internationally (> 40 different research groups), many of which are highly productive long-term relationships.
Biography
Eytan Ruppin received his M.D. and Ph.D. (Computer Science) from Tel-Aviv University where he has served as a professor of Computer Science & Medicine since 1995, conducting computational multi-disciplinary research spanning a wide variety of topics, including neuroscience, evolutionary computation, natural language processing, machine learning and systems biology. He joined the University of Maryland in July 2014 as a Computer Science professor and director of its center for bioinformatics and computational biology (CBCB), before joining the NCI in January 2018, where he founded and is Chief of its Cancer Data Science department. Eytan is a fellow of the International Society for Computational Biology (ISCB), a recipient of the NCI Director award for his work on precision oncology (2022), the DeLano award for computational biosciences for his work on synthetic lethality (2023) and the NIH director award for developing new computational paradigms for precision oncology (2024). He is a member of GSK Oncology, Pangea Biomed, Win Consortium and ProCan scientific advisory boards and a co-founder of a few precision medicine startup companies.
Related Scientific Focus Areas
This page was last updated on Friday, May 16, 2025