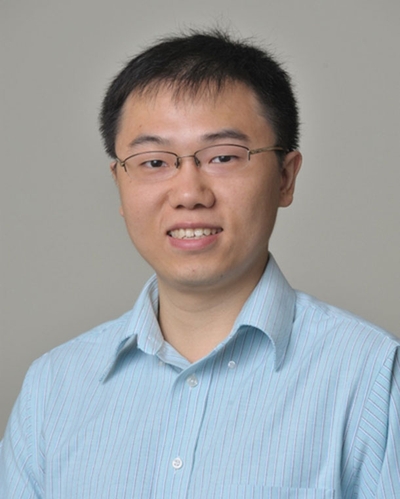
Research Topics
Dr. Danping Liu is interested in developing new statistical methods to evaluate and combine biomarkers for use in risk prediction. Individualized disease risk prediction is particularly attractive to patients, healthcare providers, and policy makers. At the population level, accurate prediction of disease helps target interventions and treatments to the most vulnerable groups. Biomarker research has attracted much attention in the diagnosis or prediction of disease outcomes, especially in studies of cancer. Particularly, Dr. Liu is interested in the following topics:
Developing methods to incorporate longitudinal biomarkers into risk prediction
It is important to incorporate longitudinal biomarkers into risk prediction of clinical endpoints, because additional information on pathology and critical time window is contained in the subject-specific trajectories of the biomarkers. Prediction accuracy could be substantially improved by incorporating the longitudinal information. Dr. Liu has been working on methods for effectively combining longitudinal biomarkers in predicting disease outcomes. In cancer risk prediction, a unique challenge is to account for the timing of, and cancer staging at, diagnosis. Dr. Liu is interested in risk prediction under a survival model framework, where the staging at diagnosis can be used to estimate the lag between cancer onset and diagnosis. While properly accounting for the timing and staging information, Dr. Liu is developing statistical methods to make formal inference on how the risk prediction accuracy of a biomarker changes over time.
Developing model selection techniques for combining high-dimensional longitudinal biomarkers
Including more biomarkers into a prediction model does not always improve prediction accuracy. Selection of markers is a crucial step, especially when the number of candidate markers is large. Marker selection involves several aspects: selecting markers with high classification power, selecting the important time points for making observations, and selecting subgroups of patients for enhanced prediction. Dr. Liu is interested in developing model selection techniques for longitudinal risk prediction.
Missing data problems in biomarker evaluation and combination
Incomplete observations are common in most longitudinal studies, but this problem has not been studied in the context of biomarker combination. Both the biomarker and the outcome could be missing due to loss of follow-up. The absence might be informative if the dropout process depends on a subject’s unobserved characteristics. For example, the subjects who develop cancer sooner have a shorter follow-up and hence fewer biomarker observations. Dr. Liu’s interest lies in developing efficient and robust techniques to correct for the bias that results from ignoring the missing data.
Biography
Dr. Liu received his Ph.D. in biostatistics from the University of Washington in 2010 and completed a two-year postdoctoral training at the National Alzheimer’s Coordinating Center, University of Washington. Prior to joining the National Cancer Institute (NCI), he served as a tenure-track investigator at the Eunice Kennedy Shriver National Institute of Child Health and Human Development (NICHD). Dr. Liu joined the Biostatistics Branch of the NCI Division of Cancer Epidemiology and Genetics in October 2017, and was awarded NIH scientific tenure and promoted to senior investigator in August 2024.
Selected Publications
- Fulton KA, Liu D, Haynie DL, Albert PS. MIXED MODEL AND ESTIMATING EQUATION APPROACHES FOR ZERO INFLATION IN CLUSTERED BINARY RESPONSE DATA WITH APPLICATION TO A DATING VIOLENCE STUDY. Ann Appl Stat. 2015;9(1):275-299.
- Foster JC, Liu D, Albert PS, Liu A. Identifying subgroups of enhanced predictive accuracy from longitudinal biomarker data using tree-based approaches: applications to fetal growth. J R Stat Soc Ser A Stat Soc. 2017;180(1):247-261.
- Liu D, Zhou XH. Covariate adjustment in estimating the area under ROC curve with partially missing gold standard. Biometrics. 2013;69(1):91-100.
- Liu D, Albert PS. Combination of longitudinal biomarkers in predicting binary events. Biostatistics. 2014;15(4):706-18.
- Liu D, Yeung EH, McLain AC, Xie Y, Buck Louis GM, Sundaram R. A Two-Step Approach for Analysis of Nonignorable Missing Outcomes in Longitudinal Regression: an Application to Upstate KIDS Study. Paediatr Perinat Epidemiol. 2017.
Related Scientific Focus Areas
Social and Behavioral Sciences
View additional Principal Investigators in Social and Behavioral Sciences
This page was last updated on Tuesday, July 1, 2025